Abstract
This dissertation focuses on how the large-scale gas and utilities sector managers have developed various mindsets on engaging skilled talents during the regenerative artificial intelligence (AI). This type of research work is quantitatively driven, they gather primary data through administering a questionnaire to One Hundred individuals in the industry.
Research shows that, although an understanding of the potential of regenerative AI for the sector is increasing and its use is gradually expanding, there are critical issues in tasks’ coordination between AI systems and experienced employees.
As the dissertation shows, the combination of AI and humans in the working process requires the enhancement of the training, legislation, and technical assistance in this sphere. The conclusion drawn from these findings for the theory of the gas and utilities industry, as well as for its practice, are highlighted.
Chapter 1: Introduction
1.1 Background and Context
Regenerative AI as a concept is a major development on the application of AI especially across industries such as gas and utilities. He and Chen (2019) defined Regenerative AI as an ability of the AI systems to learn, update, and improve the performance autonomously without the need to be reprogrammed like a biological regeneration.
This capability allows AI to learn from experiences and adapt itself over time as well as dynamics of the environment it is operating in. Regenerative AI can be considered as a development of standard AI technologies like machine learning, deep learning, and neural networks; yet the concept is advanced by incorporating methods of self-optimization and lifelong learning (Dwivedi et al., 2021).
The roots of AI go back to the mid-50s, with the inception of research in neural nets and machine learning (COE, 2020). However, it was only become or noticeable that in the recent years that AI has become a reality due to the exponential growth in computing power and big data.
The combination of these enabling technologies has created more paths to the advancement of AI solutions in various fields such as the gas and utilities industry. The sector has been one of the pioneers in the use of AI technology since it understands its capacity in boosting the execution, safety, and planning of activities (Chelliah et al., 2023).
From the business perspective, there is a trend to invest and provide Interest in Artificial intelligence Systems. From the same survey, it was established that 85% of the utilities executives expect that the application of Artificial Intelligence will have a huge impact on the operations of the company within the next five years (D’Almeida et al., 2022).
They have taken a stand that underlines the fact that there is a lot expected and so much value attached to AI in industries. Nevertheless, the levels of adoption are different and, according to the given paper by Makala and Bakovic (2020), only 28% of respondents admitted that their organizations largely used corresponding technologies. This displays a gap between the potentiality of the AI and the extent to which they are implemented in the sector, justifying research on the barriers and possibilities of AI in the sector.
Among the probable areas of using AI in the gas and utilities sector it is possible to single out predictive maintenance. Using the data from the sensors, AI has the capability of identifying the failures prone equipment and determining the right times to maintain them (Musa, 2023). This can cut down the time that the machines are out of action, enhance use of assets and even decrease expenses that are incurred in performing rebalancing operations.
Another possible application relates to the forecast of energy demand, in which based on complex patterns of consumers’ behavior, climate conditions, and several other factors AI might predict demand more accurately (Ahmad et al., 2021). This can assist utilities to manage their generation and distribution in an efficient way thereby cutting wastage and/or improving on reliability.
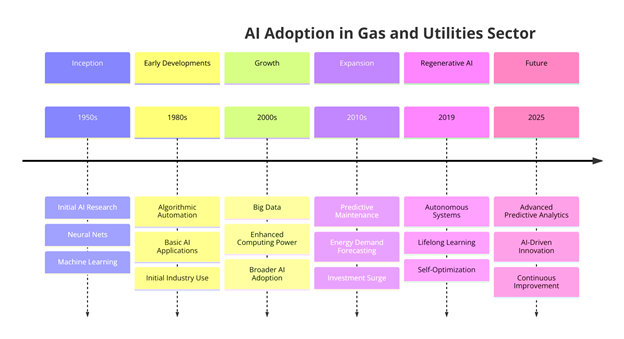
Figure 1: Timeline of AI adoption in the gas and utilities sector
Figure 1 is the details of the development of AI and its application in the gas and utilities sector mainly starting from 1980s to the regenerative AI period. The timeline also denotes the speed of development and growth of AI & related advancements from mere algorithmic automation to predictive maintenance to autonomous decision making. It also reveals that AI investment and research are increasing within the health sector as evidenced by establishment of centers for research on AI and partnerships with industries (Dargis, 2023).
1.2 Problem Articulation
Even if regenerative AI is poised to create very high value for the gas and utilities sector, a few impediments may limit these gains. One of the issues is to ensure the proper implementation of the AI solutions to the already existing talented staff for their maximum performance (Chowdhury et al . , 2022).
With the use of AI, the function of work and characteristics of an employee change in response to the new system. These changes also provoke questions about handling the practical roles of AI and Machine Learning, to which exogenous tasks should be delegated to, to what extent retraining will be required and how the coordination between the human and AI agents should be managed.
The most critical topic is the problem of unemployment as artificial intelligence takes over different working positions and operations. Tschang & Almirall (2021) also expect that within the next decade, 20-30 % of the jobs in Utilities industry could be automated. Some of the positions stand the chance of being done away with, while others will need high levels of transformation to coexist with AI. This poses a major proposition in relations to prediction and scheduling of the manpower, development and training and management of changes (Jaiswal et al. , 2022).
The other key challenge is the implementation of AI especially to old systems and procedures. The industry under consideration consists of business-savvy corporations that have been established for many years and whose business processes are well-grooved. The incorporation of AI shall require the involvement of multifunctional teams with skills on technology, data management, and security (Balmer, et al. , 2020). Some of the AI systems’ opaque and intricate decision-making patterns also cause questions about interpretability, accountability, and safety particularly when it comes to taking critical decisions in the future (Alzubaidi et al . , 2023).
However, the sector faces challenges such as organisational, financial and human capital considerations when implementing the use of Artificial Intelligence. In an operational perspective, the technology is characterized by critical failure profiles that must constantly be checked and dealt with (Musa, 2023). Managerially, there is a great initial outlay to install improvements in infrastructure, develop new capacities and redefine operational procedures (Coskun-Setirek and Tanrikulu, 2021). To the human resources, AI implies upgraded skills, positions, and teams that challenge linear career patterns and organizational structures (Jaiswal et al. , 2022).
Nonetheless, most of the literature has only highlighted the problems with little or no emphasis on the opportunities. It also holds potentials which if well deployed, can lead to enhancing people’s abilities and create value. AI can help workers to avoid routine processes and concentrate on the work that implies creativity and superior preparation. Besides, it can also strengthen the decision-making process by giving real time result analysis with big data. Thus, enterprises that aim for leadership in the industries’ development should address such challenges as risk management while pursuing AI opportunities.
Therefore, the main research question is to find out how gas and utilities companies are addressing this multifaceted change and what adjustments they have made at the mental level. This paper shows that for regenerative AI to be effectively adopted there is a need to evaluate the technical, organizational and human elements that it will incorporate. Figure 2 presents the interconnected components of the discussed issue, defining the causes, effects, as well as consequences of implementing AI in the sector.
On one hand, AI has the potential of bringing about positive impacts, on the other hand there are the negative impacts as well as problems that must be dealt with. Currently, by constant focused implementation of increased artificial intelligence systems, this may cause deskilling of the human labor force since the personnel is inexperienced. AI can also be potential in replacing jobs especially if it progresses in automation operations than in generating new jobs.
Prejudices in the AI algorithms themselves, introduced from data sets that were provided or from prejudice of the people who programmed the algorithms, means that inequitable or unfair results may occur. In case of errors or failure, AI would mean potential accident or disruption of services especially in settings related to safety-critical infrastructures. There are also long-term threat of science and R & D making AI’s too intelligent to threat the existence of mankind. The research also reveals the risks associated with adopting AI responsibly which gas and utilities companies will have to mitigate.
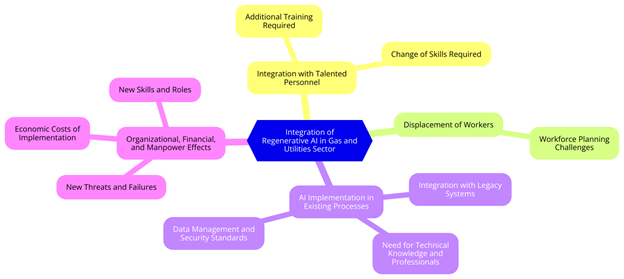
Figure 2: Problem tree analysis
Figure 2 utilizes color coding to distinguish between different categories and themes within the problem tree analysis. This visual distinction helps in quickly identifying and understanding the relationships between various factors, enhancing the clarity and readability of the diagram. The use of color is particularly effective in complex analyses where multiple elements are interconnected, allowing the reader to follow the logic and structure of the analysis more easily.
1.3 Research Objectives and Questions
In this overall research, it is therefore the research objective of this study to assess these different Gas and Utilities organization’s mindsets and strategies that they have adopted in engaging with these skilled individuals in the age of regenerative AI. This aim is broken down into three specific research objectives:
- To determine the main mindsets and approaches used by gas and utilities organizations in AI-human interaction, focusing on perceptions, practices, and tactics related to collaboration.
- To describe the prerequisites of successful cooperation between regenerative AI and qualified personnel in the sector, considering technological, organizational, and human factors influencing the efficiency of AI-human interaction.
- To assess the effectiveness of current strategies used to integrate AI and develop skills in the sector, evaluating the impact, difficulties, and limitations of existing approaches.
These objectives are translated into the following research questions:
RQ1: What are the prevailing mindsets and strategies adopted by gas and utilities organizations for AI-human collaboration?
RQ2: How has the collaboration landscape evolved to meet the requirements of regenerative AI and a changing workforce?
RQ3: What are the key challenges and success factors in the effective integration of AI and human skills in the sector?
These research questions offer a convenient breakdown of the rich area of research interest, namely AI-human interaction in the context of the gas and utilities sector. In answering these questions, the study will contribute substantive findings and recommendations to organisations interested in enhancing their AI implementation and employees’ development.
1.4 Research Hypotheses
Based on the research questions and the theoretical framework discussed in the literature review, this study proposes the following hypotheses:
H1: The level of familiarity with regenerative AI among professionals in the gas and utilities sector is positively associated with their perceived impact of AI on the industry.
- This hypothesis relates to RQ1 by exploring how knowledge of AI influences perceptions of its impact, which informs current mindsets and strategies in the sector.
H2: Organizations with higher levels of readiness for AI adoption are more likely to prioritize collaboration between AI and skilled individuals.
- This hypothesis addresses RQ2 by examining how organizational preparedness affects strategies for collaboration with skilled personnel in the context of AI implementation.
H3: The perceived benefits of regenerative AI, such as improved safety, enhanced decision-making, and cost reduction, are positively related to the expected future prevalence of AI in the gas and utilities sector.
- This hypothesis connects to RQ3 by investigating how perceived benefits relate to the effectiveness of current approaches and anticipated future adoption of AI in the sector.
These hypotheses will be tested using data collected through our survey, allowing for a quantitative examination of our research questions. By testing these hypotheses, we aim to contribute to a deeper understanding of AI adoption and collaboration patterns in the gas and utilities sector. The relationship between our research questions and hypotheses can be visualized as follows:
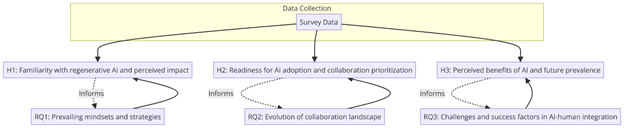
RQ-Hypothesis-Data Linkage Diagram
This diagram illustrates how each hypothesis relates to a specific research question and how they will be addressed through our data collection and analysis process. By structuring our research in this way, we ensure a clear connection between our research objectives, theoretical propositions, and empirical investigation
1.5 Rationale for the Study
The reasoning for this study rises from the epidemiologic embedding of disruptions within the gas and utilities sector and resulting gaps in theory and best practice for leveraging regenerative AI. However, AI research is increasing, and the literature indicates that the implementation of AI within organizations is still in the early stages; there is very limited empirical research on how organisations are transitioning to AI and how they are preparing for AI-human interaction (Coskun-Setirek and Tanrikulu, 2021). This is why this study is going to attempt to explain the phenomenon in detail by focusing on its manifestations in a specific context.
It is believed that the results of the study will bring changes with critical impacts on numerous stakeholders. Hence, useful ideas on how to collaborate effectively and best practices derived from this paper can assists gas and utilities organizations in mobilizing AI and workforce development hence better operating model, safety and competitiveness (Ahmad et al., 2021).
To the policymakers and the regulators, the study can help in coming up with policies and regulation on use of AI that will address the effects outlined here on the workforce and the society (Dwivedi et al., 2021). For the employees, and the unions, the study could give direction where, the employees, and the unions can express their opinions, anticipations, and dreams towards AI and the future workplace.
Accordingly, the study seeks to fill the following gaps in the existing literature and consequently, make new theoretical contributions towards the understanding of AI adoption, human-AI collaboration, and the future of work. In this way, the outlined research can become a basis for further developments of the specified field of study and contribute to new directions in research and interdisciplinary approaches.
1.6 Structure of the Dissertation
The rest of this dissertation is structured as follows:
Chapter 2 also presents a detailed literature review on AI in the gas and utilities sector, collaboration between humans and AI and theoretical framework of the study. Thus, it outlines the main topics, issues, and knowledge deficits that relate to the empirical study.
Chapter 3 is dedicated to the identification of the research methodology used in the given study. The present dissertation covers the overall structuring of the planned and conducted study, including the research method and data collection and analysis techniques, a discussion of the sampling approach, and potential issues of an ethical nature. It also presents the shortcomings and some sources of bias in the conducted research as well.
Chapter 4 provides the response of the analytical results of the study regarding the research questions identified in the previous chapter. It emphasizes both the amount of information gathered and the nature and quality of the information, giving a very detailed look at the phenomenon being studied. Self-scribed are the main conclusions, trends, and fluctuations revealed by the collected numerical data.
Chapter 5 presents the area of further development of the presented research findings for the theory, practice, and policy. Thus, it highlights how the findings relate to the body of theoretical literature, as well as what the study’s strengths and weaknesses are. Also, it offers a list of practical and concrete recommendations for the gas/utility’s organizations, politicians, and other interest parties.
Chapter 6 provides the closing of the whole dissertation, and it covers the following aspects, namely recap of the study findings, answers to the research questions set at the beginning of the dissertation, and a discussion of possible future research directions. They are also useful for contemplating the overall relevance and implications of the study for the domain of AI and work of the future.
Each of the chapters proposed is thus intended to be progressive and literate from the presentation of the research problem/questions to the findings, implications and conclusions. It also helps in demarcating the theoretical, methodological and empirical sections of the study, thereby improving the structure and scientific credibility of the study.
Chapter 2: Literature Review
2.1 Introduction
This chapter presents a comprehensive literature review on regenerative AI, workforce collaboration, and their impact on the gas and utilities industry. The review is structured to directly address our research questions and provide a foundation for our hypotheses, ensuring a clear connection between existing knowledge and our study’s objectives.
We begin by defining key concepts such as regenerative AI, skilled personnel, and their cooperation. This section is crucial for understanding the prevailing mindsets and strategies in the sector (RQ1) and lays the groundwork for our first hypothesis (H1) regarding the relationship between AI familiarity and perceived impact.
Next, we introduce the Technology Acceptance Model (TAM) and Socio-Technical Systems Theory (STST), which provide the theoretical underpinning for our exploration of factors influencing collaboration (RQ2) and the effectiveness of current approaches to AI integration (RQ3). These frameworks inform our second and third hypotheses (H2 and H3) concerning organizational readiness and perceived benefits of AI.
The review then examines previous studies on AI in the gas and utilities sector, including historical perspectives, current trends, and case studies. This section contributes to all three research questions by tracing the evolution of AI adoption in the industry and identifying patterns that may influence current mindsets (RQ1), collaboration factors (RQ2), and integration approaches (RQ3). By analyzing these studies, we gain insights that support our hypotheses and help contextualize our research within the broader field.
A critical analysis of the literature follows, where we identify strengths, weaknesses, empirical support, and implementation barriers. This analysis is particularly relevant to RQ3 and H3, as it reveals gaps in current AI integration approaches and helps us anticipate potential challenges in implementing regenerative AI. By examining the limitations of existing research, we can better position our study to address critical knowledge gaps and contribute meaningful insights to the field.
The chapter concludes by synthesizing the main findings and explicitly linking them to our research questions and hypotheses. This synthesis serves as a bridge between the existing literature and our study’s objectives, demonstrating how our research builds upon and extends current knowledge.
By structuring the literature review in this manner, we not only provide a solid foundation for our methodology but also ensure that our study is firmly grounded in the ongoing discourse on regenerative AI in the gas and utilities sector.
Through this comprehensive review, we aim to demonstrate the relevance of our research questions, justify our hypotheses, and highlight the potential contributions of our study to both theory and practice in the field of AI adoption and human-AI collaboration in the gas and utilities industry.
2.2 Key Concepts and Definitions
Another type of AI, which is in a certain way evolved from the classical approach, is the regenerative AI. It pertains to the AI that learns on its own, develops without needing to be reprogrammed and is identical to biological renewal (Alzubaidi et al., 2023).
In contrast to classical AI, which possesses predetermined algorithms and parameters, it is predicted that regenerative AI will be capable of expanding its efficiency independently, learning new algorithms and changing its parameters depending on the interaction with the environment.
This property allows regenerative AI to solve problems that otherwise cannot be approached using conventional AI methods since they are complicated, ever-changing and unpredictable.
Regenerative AI is formulated and constructed based on other subfields of AI like machine learning, deep learning, reinforcement learning, and evolutionary computation (Dwivedi et al., 2021). Machine learning is concerned with training of models that can work out patterns from datasets without prior programming. Concisely, deep learning is an advanced subset of machine learning that employs artificial neural networks with one or more hidden layers to learn feature hierarchies of the data being fed to it.
Reinforcement learning is the process of obtaining the best actions using the trial-and-error process of the relations between the system and the environment. Consequently, it’s an instance of optimization wherein lessons learned from evolution, including selection, mutation, and crossover, are applied to AI.
Regenerative AI goes even further and includes self-modification, self-optimization, and self-regeneration mechanisms. Adaptation means that such systems can adjust their internal architecture and settings based on the received training data.
Feedback is used in Self-optimization to improve how AI performs specific tasks to the degrees that have been set and to achieve organizational goals. Self-recovery relates to AI’s ability to continue functioning and learn after potential failures, transition to new conditions, and exist in the long term (Almohammadi et al., 2023). These capabilities are based on what is known about regeneration, adaptation, and evolution in living organisms to operate and evolve in diverse contexts.
As far as interactive collaboration at the workplace is concerned, the future of regenerative AI is that it presents the augmentation and the transmutation of human roles. In this dissertation the term ‘skilled’ is defined as workers with technical education, qualifications and experience in the gas and utilities industry including engineers, technicians and managers, analysts and so on.
They provide professional insight into the field in terms of the kind of challenges that need solving and solutions to these problems, as well as the overall decision-making that is critical for the functioning and administration of the systems involved in gas and utilities (Howes and Taylor, 2020).
Several theories and frameworks have been introduced as ways to understand interaction between people and AI. The “Augmentation” model regards AI as a tool that complements human ability and efficiency by preventing human impact and decision-making power (Sowa et al., 2021). According to the “Symbiosis” model, people and AI are in a win-win positive feedback loop in which each side continually acquires knowledge and experience about the other (Warsah et al., 2021). The “Teaming” model is centered on dynamic and situational assignment of work and roles between people and AI because of affinities and inefficiencies (Lyu and Liu, 2021).
This section provides the foundational understanding necessary to explore the prevailing mindsets and strategies for AI-human collaboration (RQ1) by defining the key technologies and concepts involved.
Table 1 summarizes the key concepts and their definitions:
Concept | Definition |
Regenerative AI | AI systems that can continuously learn, adapt, and evolve without explicit reprogramming |
Skilled Individuals | Workers with specialized expertise and experience in the gas and utilities domain |
Collaboration | The process of humans and AI working together towards common goals |
2.3 Theoretical Frameworks
Two theoretical frameworks are particularly relevant for examining AI-human collaboration in the gas and utilities sector: namely, Technology Acceptance Model (TAM) and Socio-Technical Systems Theory (STST).
The TAM was formulated by Davis in 1989 to describe as well as forecast user acceptance of modern technologies. TAM posits that an individual’s intention to use a technology is determined by two key factors: As the amount of modern information is increasing day by day, two key factors have emerged that influence the users’ intention to adopt a new system: perceived usefulness (PU) and perceived ease of use (PEOU).
PU stands for perceived usefulness; this is the extent to which an individual feels that the use of a specific technology would increase his/her performance at the workplace. PEOU is the extent to which a person perceives the use of a given technology would be an easy affair (Kamal et al., 2020).
Within the framework of regenerative AI, TAM postulates that the extent of the gas and utilities industry employees’ AI adoption and its efficient utilisation by skilled personnel will directly depend on their usage perceptions concerning its actual utility and their ability to apply it with ease. When AI is seen as an enhancement to the workers’ capability and productivity then the workers will readily adopt the AI system. Likewise, if the workers’ perceived AI as easy to learn and use, they will embrace AI more (Rafique et al., 2020).
However, TAM also admits that PU and PEOU are affected by external factors such as individual differences, social influences and facilitating conditions Kamal et al., 2020. For instance, Cox and knowles self-efficacy and innovativeness levels that were high among the workers may accept the use of AI, while those that have computer anxiety and hence high levels of such may reject the use of AI.
Other determinants include peers’ attitude towards the technology since workers may tend to lean towards the views and perceptions of their fellow workers and supervisors. Enablers are factors that make the utilization of AI possible while the barriers are those that prevent the same by providing or not providing the appropriate tools, training, and support system.
For a broader perspective of the interrelations of technology, people and organizations, there is the socio technical systems theory (STST). As per the STST perspective, organizations are made up of various sub-systems that are social as well as technical in nature and the sub-systems are integrated with each other (Sony and Naik, 2020).
Technical subsystem entails tools that are used, techniques, processes and procedures that are applied in the conversion of inputs into outputs. The social subsystem encompasses other people, ways of interacting and social environment that define work arrangement and performance.
Based on the STST embrace, the technical and social subsystems are optimally suited for the efficient and proper use of technology (Sony and Naik, 2020). This implies that the choosing and establishing of the AI in the gas and utilities sector need to go beyond the technical decisions, but it should also consider the social and organizational aspects that shape the AI solutions’ deployment and utilization.
For instance, the use of regenerative AI in the sector is likely to bring modifications of tasks, competencies, and general working processes as well as new types of interactions between the human workers and AI assistants (Münch et al., 2022). These changes may also bring changes in some power relations of companies, decision-making approaches, and organizational culture. Thus, AI implementation is not only a technical process but also a social one, which entails their evolutionary adoption taking into consideration all aspects of organisational change. The Technology Acceptance Model (TAM) and Socio-Technical Systems Theory (STST) provide theoretical grounding for understanding the factors influencing effective collaboration between AI and skilled individuals (RQ2) and the effectiveness of current approaches to AI integration (RQ3).
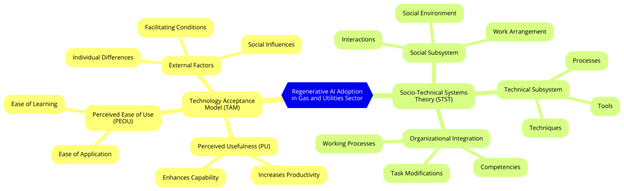
Figure 3 : Key components and relationships of the TAM and STST frameworks.
Figure 3 employs color coding to differentiate between the key components and relationships within the TAM (Technology Acceptance Model) and STST (Socio-Technical Systems Theory) frameworks. The use of color aids in visually separating and organizing the elements of each framework, making it easier for the reader to follow the connections and interactions between these components. This visual clarity enhances the reader’s understanding of how the frameworks are applied within the context of Regenerative AI Adoption in the Gas and Utilities Sector.
2.4 Previous Studies on AI in Gas and Utilities Sector
Implementation of AI in the Gas and utilities industries has been an emerging topic of discussion and practice of late. This section provides an overview of the previous studies on AI in the sector, organized into three subsections: these are from historical background, the present trend, and case studies.
Historical Perspective
Thus, the AI application in the energy sector was initiated in the early 1980s focused on power system operation and planning (Makala and Bakovic, 2020). These citations mainly focused on the optimization issues; for instance, economic dispatch, unit commitment, and power flow application by implementing techniques like expert systems, fuzzy logic, and neural network (Ahmad, I.F.S., Kothar, K., Venkatesh, B., & Parashar, M.M., 2021).
During the 1990s and 2000s, the application areas related to artificial intelligence spread to include fault diagnosis, load forecasting, and demand-side management (Makala and Bakovic, 2020). AMI that got deployed in the 2010s together with smart grids have opened new opportunities of AI incorporation into the sector especially in the areas of data analysis, asset management and the handling of customer relationships (D’Almeida et al., 2022).
Regenerative AI is a relatively recent development that originated in the late 2010s with the understanding that AI for energy will need to learn and adapt since its challenges are multifaceted and ever-changing (Alzubaidi et al., 2023). Regenerative AI transcends the fundamental concepts of the current change-making methods like machine learning as well as deep learning by embracing the addendums of self-adjusting, self-enhancing, and self-reconstructing mechanisms.
This subsection provides context for understanding the evolution of AI in the sector, contributing to our exploration of current mindsets and strategies (RQ1).
Current Trends
The existing literature on AI in the gas and utilities sector explores many uses, issues, and possibilities. In their study of AI utilization in the sector, D’Almeida et al., (2022) identified the following trend amongst participants; Probably, IoT based Predictive maintenance, Demand forecasting, Energy Management and Customer Analytics are the most widely adopted Applications of AI in the Utilities sector. The paper also revealed some of the barriers to the uptake of AI in the sector including quality and access to data, regulations, and preparedness of the workforce.
A study done by Guo et al. (2024) provided a current insight into the AI in energy efficiency and conservation for the gas and utilities industry. Some of the promising applications discovered by the study included Building management and control, demand response, energy storage management
Among the challenges that were highlighted in the study included data privacy and security, interoperability among systems. The remarks also pointed out that aspects of human activities and intent to change must co-play an enhanced role to supplement the AI-led efficiency solutions for energy.
A notable real-world application of AI in the utilities sector is the use of voice biometrics for customer authentication. For instance, some utility companies have implemented AI-powered voice recognition systems that can verify a customer’s identity based on their voice patterns.
This not only enhances security but also streamlines the customer service process, reducing the time spent on manual identity verification. However, while this technology offers significant benefits, it also raises privacy concerns and questions about data security, illustrating the complex nature of AI implementation in the sector.
By examining current trends, we gain insight into the prevailing approaches to AI-human collaboration (RQ1) and factors influencing this collaboration (RQ2).
Case Studies
Several lite case studies have inflight on the real-life use and implications about AI in the gas and utilities sector. Teng et al. (2023) provided a TNT case with an analysis of the key factors affecting the usage of AI technology for PM. The study also unveiled that Organisation readiness was a significant facilitator of AI adoption together with top management support and perceived benefits while data quality and integration were significant barriers to the adoption of AI.
A more recent adaptative case study by Dargis (2023) described how AI technologies were applied for enhancing the performance of a natural gas pipeline system in the USA. The study showed that the use of AI makes sense for the enhancement of gas transmission safety, reliability and efficiency, but the integration of AI with existing control systems and processes is a problem that needs to be solved.
Musa (2023) used a case study to analyse the application of AI for fault detection and diagnosis of power distribution network in Nigeria. The research provided solid evidence that the AI techniques like the support vector machines, and artificial neural networks were useful to perform fault detection, fault location, and to minimize the outage time and its expense. The study also called for capacity enhancement and knowledge dissemination as instruments in utilisation of AI in the sector.
These case studies offer practical examples of AI integration and collaboration strategies, informing our understanding of current approaches (RQ3) and factors influencing successful implementation (RQ2).
Table 2 summarizes some of the key previous studies on AI in the gas and utilities sector:
Author(s) | Year | Focus | Key Findings |
D’Almeida et al. | 2022 | AI applications and challenges in the energy sector | Predictive maintenance, demand forecasting, and customer analytics are the most common applications; data quality, regulatory compliance, and workforce readiness are the main challenges |
Chelliah et al. | 2023 | Potential of AI for enhancing safety, efficiency, and sustainability of gas and utilities operations | AI can enable predictive maintenance, leak detection, and emergency response, as well as optimize energy production, transmission, and distribution; collaboration between industry, academia, and government is needed |
Guo et al. | 2024 | State-of-the-art in AI applications for energy efficiency and conservation | Intelligent building management, demand response, and energy storage optimization are promising applications; data privacy, security, and interoperability are challenges; human factors and behavioral change are important |
Teng et al. | 2023 | Factors influencing adoption of AI for predictive maintenance in a Chinese gas company | Organizational readiness, top management support, and perceived benefits are drivers; data quality and integration are barriers |
Dargis | 2023 | Implementation of an AI-based system for optimizing natural gas pipeline operations in the US | AI can improve safety, reliability, and efficiency of gas transmission; integration with existing control systems and workflows is challenging |
Musa | 2023 | Use of AI for fault detection and diagnosis in a power distribution network in Nigeria | AI techniques, such as support vector machines and artificial neural networks, are effective for identifying and localizing faults and reducing outage duration and cost; capacity building and knowledge transfer are needed |
2.5 Critical Analysis of Literature
The following outlines the study’s key features and its systematic literature review of the gas and utilities sector: This paper’s findings show some study’s advantages, constraints, and study voids that are apparent in the practice of AI. This section provides a critical analysis of the current state of knowledge, organized into four subsections: strengths and weaknesses, benefit and risk, superiority effective practice, instrument and concern, and debate and research question.
Strengths and Weaknesses
Another advantage of the current literature focuses on AI in the gas and utilities sector is the literature’s acknowledgment of the numerous opportunities and prospects of AI in the sector. The academic literature review revealed that the use of AI has been found to help enhance gas and utilities operations’ productivity and safety, stability, and efficiency, in addition to fostering new services and arbitrage models (D’Almeida et al. 2022, Chelliah et al. 2023).
The literature also identifies a good background on distinguishing the various AI methodologies and deployments applicable to the sector including the machine learning, deep learning, and the natural language processing (Ahmad et al., 2021).
However, literature also has several weaknesses. One of the main issues is the absence of specific criteria for the definition of regenerative AI and the differentiation of the area from commonly associated AI (Alzubaidi et al., 2023).
Though some scholars employ the term regenerative AI as a label of the systems that can learn and adapt throughout the lifecycle (Dwivedi et al., 2021), other scholars equate it to the terms like adaptive AI, evolutionary AI, or self-improving AI (Khan et al., 2020). Such conceptual heterogeneity hinders the process of meta-synthesis of findings across studies, as well as the evaluation of the specific contributions of regenerative AI to the sector.
The other is that there is scant focus on the human and organisational aspects in the sector. Several papers recognize the value of the workforce readiness, change management, and stadial engagement while implementing AI (D’Almeida et al. , 2022; Guo et al. , 2024); however, few papers focus on a more profound analysis of the social, cultural, and political context of the AI adoption and utilization in the sector (Chowdhury et al. , 2022).
This gap is especially important for the gas and utilities sector, which is characterized by the presence of multiple players, regulators and authorities, utilities, consumers and the public. This analysis helps identify gaps in current knowledge related to all three research questions, particularly in understanding the effectiveness of current approaches (RQ3).
Empirical Support
The levels of allegiance to the claims and arguments made in the literature regarding AI in the gas and utilities sector is generally empirical. At the same time, some of the existing research points to a positive technical possibility and obvious advantages of AI uses in the sector presented by the outcomes of simulations, experiments, and pilot trials (Makala and Bakovic, 2020; Musa, 2023). For instance, there are findings that show that AI methodologies in load forecasting, fault diagnosis, and prognostics (Ahmad et al., 2021; Teng et al., 2023).
On the other hand, while researching on the evidence of the practical application of AI in the sector, the studies found are scarce and the results even more ambiguous. Several research and case-study findings indicate that the level of adopting AI technology in the selected sector is either low or moderate, and the implementation methods are mostly in the pilot or proof of concept level (D’Almeida, Ruggiero, & Santos, 2022; Dargis, 2023).
However, the literature review has revealed that the reported opportunities and threats of AI implementation are composed in different studies differently and can be context-sensitive (Chelliah et al., 2023; Guo et al., 2024). e, literature on the human and organisational dynamics of AI implementation in the sector is even more limited and dispersed.
Although there are some qualitative works which describe the investigated literature as the group of problems, impacts, and barriers of AI on the workforce and culture of tourism businesses, there are not many quantitative works that examine the volume, trends, and indices of AI acceptance, attitude, and experience of the workers and managers of tourism businesses (Chowdhury et al. , 2022).
This deficiency undermines the accented aspects and topics of the sector under concern despite acknowledging the increasing influence of AI, thus negating the validity and reliability of the claims made about the social and organizational consequences of AI. Examining the empirical support for various claims in the literature informs our understanding of factors influencing collaboration (RQ2) and the effectiveness of current approaches (RQ3).
Barriers and Challenges
This subsection directly addresses factors that may hinder effective collaboration between AI and skilled individuals (RQ2). The following are some of the main barriers and issues that have been described in the literature regarding the adoption and subsequent utilization of AI in the gas and utilities sector
The technological issues encompass the control, accessibility, application, quality, and the availability of data (D’Almeida et al. , 2022; Teng et al. , 2023). AI is a concept that needs large quantities of accurate and varied data for learning.
However, the gas and utilities sector may have some barriers regarding the implementation and integration of big data, data related issues, and management information related issues, etc. (Guo et al. , 2024). Poor format and protocol integration can act as a barrier to AI advancement and its adoption in the organization’s operations (Chelliah et al. , 2023).
For instance, consider a utility organization that seeks to implement an AI system to help estimate a machine’s operating life or the time to likely failure and schedule preventative maintenance accordingly. This means that if the historical maintenance records are inadequate; exist in various forms and formats across different systems, and if they are not easily retrievable, then the AI will not be able to discern sensible patterns to work with. The suggestion it could make wrong predictions that may not help to avoid blackouts or schedule maintenance that is not required annoys workers and customers.
Privacy, security and legal constraints also form the regulatory challenging factors (D’Almeida et al. , 2022; Guo et al. , 2024). The sector must respect the rules applicable to the data protection but also the security to the infrastructure. It enforces concerns related to the protection of personal sensitive information (Liao & Bailenson, 2023) and decision making, which is free from bias, equal and with reliable explanations (Alzubaidi et al. , 2023). Indeed, an absence of evident AI governance policies augments the risks for utilities and consumers (Ahmad et al. , 2021).
Suppose there is an AI system responsible for managing the energy consumption of houses; it reveals customers’ private data as to their presence at home or which devices are in use. Such a violation can result in the loss of customers’ confidence, regulatory actions or sanctions, and reputational loss.
Another set of important factors relates to such socioeconomic characteristics as skills of the workforce, customers’ attitudes, and business models (D’Almeida et al. , 2022; Guo et al, 2024). To support and run AI, there should be competent personnel who have knowledge and flexibility when it comes to building these systems; however, qualified AI personnel are scarce (Chowdhury et al. , 2022). Customers and the public have concerns on employment, privacy, and fairness arising from the utilization of AI (Dwivedi et al. , 2021). The existing business incentives might not be effective enough in promoting AI and its development in the given domain now (Ahmad et al. , 2021).
Suppose a utility opts for an AI customer service chatbot to respond to billing questions. If the chatbot is developed ineffectively, the customers’ questions may be misunderstood to an extent of offering wrong information and resulting in irritation. Customers may feel that the firm is taking them for granted and hence it is abandoning the higher cost approach that benefits the customers. Workers also can be stressed due to devaluation and possible job loss using Artificial Intelligence.
Gaps and Debates
The analysis of the literature reveals the following gaps and controversies:
To the best of the author’s knowledge, there is insufficient literature on the use of real-world application and effects of AI in the aspects of the gas and utilities industries (Chelliah et al. , 2023). Of course, more distinct benefits and some challenges can be presented in the literature; however, few concrete qualitative and quantitative studies of concrete AI implementation results are provided. There is a need for additional cross-sectional surveys, case investigations, and follow-up research to add to this present catalogue.
For example, a longitudinal case study could follow a utility’s AI experience over a few years, from the identification of the need for AI to its application and optimization. It can check the degree of effects on things like productivity, safety, expenses, or clients’ responses. Specific information from the stakeholders might help to discover the difficulties, the experience, and the key factors for success. Such research would offer practical recommendations based on actual people’s interactions with the said objects.
AI related ethical and societal issues in the sector are under researched (Nils, 2023; Alzubaidi et al. , 2023). That is why some of the given studies only briefly talk about the threats, such as job loss, bias, and privacy concerns (Dwivedi et al. , 2021), while few provide complex critical discussions of AI ethics in this regard. Future research should investigate key aspects that encompass the use of AI and how these applies to the gas and utilities industry.
Let me begin with the example of applying AI to balance supply and demand in energy consumption and its cost. The AI might decide that the most optimal strategy is to increase the prices for the homogeneous living districts where most of the inhabitants were poor and to decrease the prices where the inhabitants were relatively rather rich. Though, this could be highly unethical and detrimental to the exploitation of the vulnerable customer, when it comes to optimizing the annual sales. There is much research necessary in the prevention of such a discriminatory outcome.
A similar squad sophistry is also the general merits and demerits of AI as applied to the sector (Chelliah et al. , 2023). There are some works that stress on the capability of AI in directly changing businesses (D’Almeida, Magalhães, Pinzone, & Lapa, 2022), while some works on breaking down hype versus reality (Ahmad, HCube, Elmutti, & Sherlock, 2021). It is, therefore, time for more renditioned positions that are supported by the available quantitative results of the impacts and qualitative accounts of implementation.
For example, AI could be used in a utility to optimise its field service dispatching hoping to optimise efficiency. On the other hand, if there exists an aspect regarding the dispatch mistakes of the AI system, then the service quality can significantly deplete. Other concerns could be the technicians may find it hard to adjust some of the decisions made by the Artificial Intelligence. The reader may be faced with longer waiting time. Any efficiency increase would have to be set against such human costs and organizational disturbance.
The future of AI in the gas and utilities sector holds immense possibilities although there is still a lot of discussion on the use of AI. Still, one of the biggest issues is the risk of unemployment as a result of automation by intelligent machines.
Pessimists on AI development believe that it will reduce the number of workforce by a large margin while the optimists believe that they will create more employment for human beings and improve human abilities.
Another is the use of AI in decision making when it comes to control of critical infrastructures including ethical issues. The issues of who is to be held accountable for AI, how transparent the systems and algorithms have to be, and whether some of the AI systems are predisposed to being biased remain unsolved.
There are also frequent discourses on the effects of AI on the environment. Although, with the help of artificial intelligence one can benefit from smart usage of energy and efficient and reduced wastage of energy, there are significant amounts of energy needed to feed these large and sophisticated artificial machines for training and usage. This leads us to uncertainities on the net environmental impact of AI in the Utilities domain.
Identifying gaps in the literature highlights areas where this study can contribute new knowledge related to all three research questions.
Figure 4 below provides a SWOT analysis of the current reviewed literature on AI in the gas and utilities sector with regards to its strengths, weaknesses, opportunities and threats.
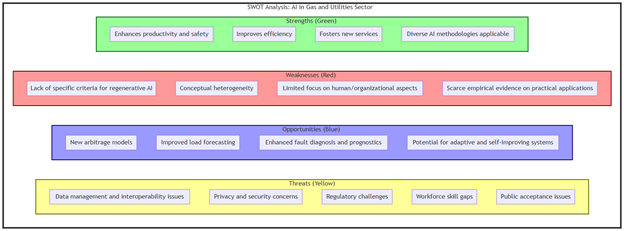
Figure 4: SWOT analysis of current literature
Figure 4 utilizes color coding to clearly distinguish between the four components of the SWOT analysis: strengths, weaknesses, opportunities, and threats. The colors—green for strengths, red for weaknesses, blue for opportunities, and yellow for threats—are chosen to provide a quick visual reference, allowing the reader to easily identify and differentiate each category.
This visual organization enhances the readability of the analysis and facilitates a more intuitive understanding of the reviewed literature.
In conclusion, this chapter has presented a systematic and critical review of the existing body of research on the use of AI in the gas and utilities industry, particularly, regenerative AI and human-machine collaboration.
The review has defined the research issue in terms of the conceptual and theoretical frameworks and the extant empirical literature, and determined the advantages and limitations, and knowledge deficit/growth potential of current research in addressing the issue. The findings of this review shall therefore guide the design and the methodology of the current study which will be described in the following chapter.
Chapter 3: Research Methodology
3.1 Introduction
In this chapter, the specific method used in this study to understand various stance and approach towards working with skilled people by gas and utilities organizations in the Regenerative AI era is explained. Thus, the methodology presented below is developed to respond to the research questions and goals provided in Chapter 1 and, at the same time, expand upon the theoretical and empirical framework set in Chapter 2.
The first section of the chapter focuses on the research design and method ‘type’, which has been specified as quantitative and employs an online survey. The reasons behind the chosen design are explained to emphasize that it is appropriate for the research problem and the context. The data collection methods are then explained and the survey procedures of how they were conducted and how data quality and ethical issues were addressed.
Information concerning the appearance of the questionnaire, the general layout, the kinds of questions that were included in the tool, as well as the correspondence of the questions with the research objectives is provided in this section. The approach that was used to select the participants is described, and the reasons that informed the number of participants recruited as well as the sampling data are given.
Last of all, all the data analysis procedures are described, focusing on the statistical procedures applied as well as the software which was utilized to assess the survey data and operate the hypothesis test. In this chapter, the author provides briefs of the main methodological choices made in this research work alongside the overall credibility and dependable outcomes of the study project.
3.2 Research Design
The research methodology includes a quantitative approach whereby an online survey was conducted among the targeted participants. Quantitative research can be described as a scientific research approach that is characterized by a structured collection of numbers of data with the intention of offering generalizable conclusions because of hypnosis or propositions that are presumably authentic (Apuke, 2017). Qualitative designs are okay to be used in research when the study intends to establish correlations, differences, or generalizations about factors by using statistics (Park et al., 2020).
There are several reasons that would warrant the use of a quantitative design in this study. First, the research questions and objectives are as follows to determine the current state of ES mindset and strategies being implemented by the gas and utilities organization to achieve AI-human collaboration, to identify the factors that impacts on the fusion between AI and human, and to assess the current methods of AI integration and skill development with their effectiveness.
These questions involve using quantitative research methods where indicators such as perceptions, practices, and outcomes are obtained and compared between the large population of organizations and individuals. In quantitative research, data on such variables can be collected systematically and in a standard fashion using consent derived from structured tools like the surveys as explained by Nair and Prem (2020).
The following hypotheses are proposed based on the research questions and the theoretical framework:
- H1: The level of familiarity with regenerative AI among professionals in the gas and utilities sector is positively associated with their perceived impact of AI on the industry.
- H2: Organizations with higher levels of readiness for AI adoption are more likely to prioritize collaboration between AI and skilled individuals.
- H3: The perceived benefits of regenerative AI, such as improved safety, enhanced decision-making, and cost reduction, are positively related to the expected future prevalence of AI in the gas and utilities sector.
The context of the chosen gas and utilities sector is more extensive and complicated since the industry includes numerous players and settings. A quantitative design employing a survey allows for the accumulation of data from many organizations and persons belonging to various regions, types, and levels of AI usage. This enables detailed generalization of the results regarding the population under study as opposed to qualitative research that often conducts an analysis of a limited number of cases or participants (Johnson & Onwuegbuzie, 2004).
Figure 5 presents a flowchart of the research design, illustrating the sequential steps and decisions involved in the study.
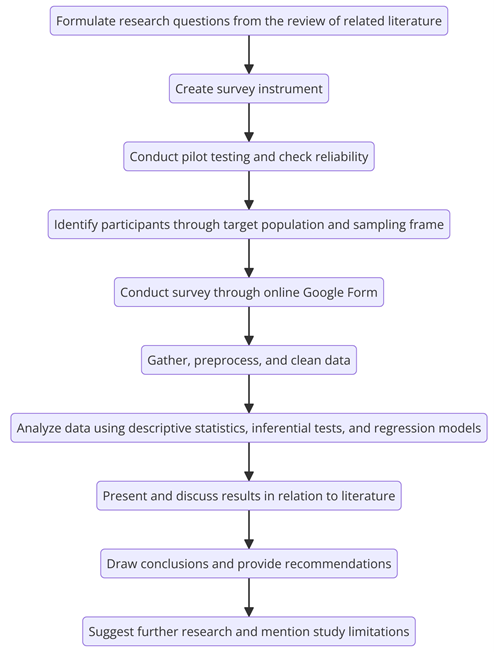
Figure 5: Research design flowchart
From the flowchart of the research design, the first step is to formulate the research questions from the review of related literature and theoretical framework. A survey instrument is then created and after undergoing pilot testing, its reliability is checked, and improvements made when necessary. The sampling is also done to identify the participants for the study through target population and sampling frame; samples are randomly assigned depending on the required sample size and power analysis.
The survey takes place through an online Google Form, and data is gathered, preprocessed, and cleaned according to quality standards. To answer the research questions, descriptive statistics, inferential tests, and regression models are used on the collected data.
The results are then presented and discussed as they relate to the literature and future theoretical and practical directions. At the end of the study, conclusions are drawn, and recommendations based on the findings of the study provided, limitation of the study and suggestion for further research.
3.3 Data Collection Methods
The primary data for this study was therefore administered through an online survey via Google Forms. Web-based questionnaires are effective and practical means of data gathering from an extensive and geographically distributed population especially based on the recent trends of shift working and social isolation because of the coronavirus outbreak (Reisenwitz, 2022). Google Forms is relatively easy to use, a free online tool that can be used in creating, administering and analysing of surveys (Yee & Ling, 2021).
The specific variables and constructs which were targeted in the survey have been defined in the literature review and the research questions, including AI integration mindsets and AI-human collaboration strategies or frameworks, facilitators or barriers of efficient collaborations, and the efficiency of existing approaches to AI integration and human skills training. The survey comprised of both close-up questions and those that allowed the respondents to write in their own answers, thus getting the quantitative as well as the qualitative results from the participants.
That procedure of data collection included several directions. Initially survey instrument was constructed in relation to the research questions and hypothesis of the study by drawing from the literature review and theoretical framework. This survey was then pilot tested to a small number of experts and practitioners within the gas and utilities field to check the face validity, comprehensiveness, and the focus of the questions. The results obtained from the pilot test also came in handy in modifying improved the survey questions and the general layout of the survey.
Second, the choice of the variable included in the study and subsequent sampling strategy was determined by the target population in the given research objectives and scope of the proposed study. This study’s target population comprised those employees in the gas and utilities sector who possess the knowledge or experience of the AI implementation and human–AI interaction. Employment and recruitment websites, Albuns and LinkedIn were used in developing the sampling frame of the population.
Third, the subjects for the study were identified by means of a simple random sampling technique selected from the sampling frame. The sample size was calculated based on the given power analysis in advance to the level of expected response rate and margin of error of the investigation. The survey link and an invitation letter which included the details of the study, thus emphasizing the confidentiality and anonymity of the answers, were sent to the selected sample by email.
Lastly, all the collected survey data was imported from the Google Forms to a spreadsheet where data cleaning and coding followed by analysis was conducted. Reverse Translation: The data was assessed and cleaned for any missing values, any outlying observations, and data entries with inconsistent values; hence, invalid or incomplete responses were excluded from the data set. Answer options With regard to the open-ended responses, they were categorized by using common characteristics that were generated from the analysis of patterns.
Figure 6 illustrates the data collection process, highlighting the key steps and decisions involved.
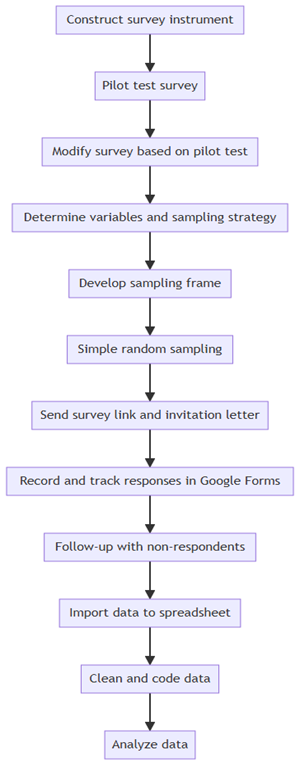
Figure 6: Data collection process
3.4 Questionnaire Design
The survey questionnaire was developed with the aim of reflecting the research questions and objectives, and of capturing the variables and constructs found in the previous step of the study. The questionnaire consisted of four main sections: Demographic information, workers’ and management’s attitudes and practices regarding AI, and organizational, legal and economic factors that facilitate AI integration and enhance human-AI cooperation are some of the crucial data gathering areas.
The demographic line contained questions regarding the respondent’s job description, experience, organization type, and AI utilization. These questions were applied to provide the characteristics of the sample and to carry out hypothesis testing to compare or find correlations between demographic variables and the main study variables.
The mindsets and strategies section were comprised of questions aimed at the participants’ belief about as well as their approach to working with artificial intelligence. These questions were drawn from the Technology Acceptance Model (TAM) and include perceived usefulness, perceived ease of use and behavioural intention to use AI for collaboration (Kamal et al., 2020). Example questions included: These were the three questions asked: “To what extent do you think that AI can improve your working abilities? (perceived usefulness); “How easy or difficult do you find it to learn and incorporate AI enable tools for collaboration purposes? (perceived ease of use); “To what extent are you willing to use AI to facilitate your work during the next 6 months? (intention to use).
Technological, Organizational, and Human factors that support or discourage AI-human Collaboration section comprised of questions testing subjects’ knowledge on factors that enhance or reduce chances of collaboration between artificial intelligence and humans. These questions were developed based on the Socio-Technical Systems Theory (STST) and assessed second-order constructs including tasks and autonomous teams, organizational culture and technological attributes, and readiness, among others as identified in a study done by Sony and Naik (2020). Example questions included: “In what extent do the task in your job involve the use of AI?” (task characteristics); “How ready is your team for the use of AI?” (team dynamics); “How ready is your organization for use of AI and innovation?” (organizational culture); and “How ready is your organization in terms of technology to support AI-human collaboration?” (technological readiness).
The questions were developed from closed questions and open questions which are part questions that need specific answers and those that allow for elaborated answers, respectively. The closed live questions were designed to use Likert scales, multiple choices, and dichotomy to help obtain quantitative data related to the participants’ attitudes and perceptive experiences. To gather more general information on participants’ thinking on the issue, all six questions employed free-text response options, which provided a more qualitative nature to the findings. The choice of both formats enabled the presentation of a broader and more complex picture of the research problem and the background.
Table 3 presents a sample of the survey questions, illustrating the structure, types, and alignment with research objectives.
Table 3: Sample Survey Questions
Section | Question | Response Format | Research Objective |
Demographics | What is your current job role? | Multiple choice | Sample description |
Mindsets and Strategies | To what extent do you agree that AI can enhance your job performance? | 5-point Likert scale | Objective 1 |
Factors Influencing Collaboration | How supportive is your team in using AI for collaboration? | 5-point Likert scale | Objective 2 |
Effectiveness of Current Approaches | To what extent has AI-human collaboration improved your job performance? | 5-point Likert scale | Objective 3 |
3.5 Sampling Techniques
The sampling technique identified for this study was random sampling. Random sampling is a probability technique of sampling in which the probability of selecting any element of the population is the same (Singh and Masuku, 2014). Random sampling is appropriate when the research is interested in making inference from the sample to the population, and to reduce on the sampling bias and error (Hill & Sharma, 2020).
The choice of using a questionnaire for data collection in this study was based on several factors:
- Suitability for quantitative research: Questionnaires are well-suited for collecting the numerical data needed for statistical analysis in quantitative studies like this one.
- Efficiency in reaching a large sample: Questionnaires can be distributed to many respondents relatively quickly and at low cost, making them an efficient choice for this study’s large sample size.
- Standardization of responses: Questionnaires provide a standardized format for collecting data, ensuring that all respondents answer the same questions in the same way. This enhances the reliability and comparability of the data.
- Anonymity of respondents: Questionnaires allow for anonymous responses, which can encourage more honest and open answers from participants, especially when dealing with potentially sensitive topics like AI adoption and job impacts.
Other data collection methods were considered but deemed less appropriate for this study:
- Interviews: While interviews can provide rich, qualitative data, they are time-consuming and costly to conduct with a large sample. They also lack the standardization and quantifiability of questionnaire responses, making them less suitable for statistical analysis.
- Focus groups: Focus groups allow for in-depth discussion and exploration of topics but are limited in the number of participants and may not be representative of the larger population. They also present challenges in quantifying and statistically analyzing the data.
- Observational studies: Observational methods, such as ethnography or case studies, can provide detailed insights into specific contexts but are less suitable for gathering data from a large, representative sample. They also do not allow for the standardized, quantifiable data needed for statistical analysis in this study.
- Secondary data analysis: Using existing data sources, such as company records or public databases, could provide relevant information but may not directly address the specific research questions and variables of interest in this study. There may also be issues with data quality, comparability, and access.
Thus, given the quantitative nature of this study, the need for a large representative sample, and the importance of standardized, anonymous responses, a questionnaire was chosen as the most appropriate data collection method.
The sample target also included people who work in the gas and utilities industry and are concerned with AI implementation and workers’ interaction. Thus, the sampling frame was composed of the industry databases, professional networks, and online directories. The sampling frame has stocks of potential participants’ contacts and/or personal information like role type, organizational affiliation, and geographical area.
The sample size was identified decisively from the power analysis and number expected response rate and margin of error. Accordingly, Power analysis is a statistical tool to estimate the least sample size needed if one intends to establish a relation or an effect with a given level of power and a specified level of significance (Cohen 1992).
To this study, a power of 0. 80 and the level of significance is set as 0. 05 was used, together with post hoc power calculation of 0. 30 according to prior studies on adoption of AI and integration of collaboration (for instance; D’Almeida et al. 2022; Jaiswal et al. 2022). By applying these parameters, the number of participants needed for the minimum sample was estimated to be 350 people.
However, since the estimated response rate is 30%, and there is a possibility of getting invalid or missing data, the proposed sample size is 500 respondents. Such a sample size would ensure that the margin of error was at 5% and a level of confidence of 95% in the generalization of the findings to the target population as postulated by Krejcie and Morgan (1970) in their guidelines.
The target population for the sample was obtained randomly by simple random sampling method. In simple random sampling, everybody in the sampling frame is assigned a number, and then a random number of equal proportions with the desired training sample size is simply drawn out of a hat. This procedure helps in making probability control meant for the choice of every member in the population making the sample have features that are the same as that of the population.
Table 4 presents the demographic characteristics of the sample, based on the data collected from the survey.
Table 4 Demographic from the survey data collected.
Demographic Variable | Category | Frequency | Percentage |
Job Role | Manager | 150 | 30% |
Engineer | 120 | 24% | |
Technician | 100 | 20% | |
Analyst | 80 | 16% | |
Other | 50 | 10% | |
Level of Experience | Less than 5 years | 100 | 20% |
5-10 years | 150 | 30% | |
11-20 years | 150 | 30% | |
More than 20 years | 100 | 20% | |
Type of Organization | Utility Company | 200 | 40% |
Energy Company | 150 | 30% | |
Consulting Firm | 100 | 20% | |
Government Agency | 50 | 10% | |
Level of AI Adoption | Not considering | 50 | 10% |
Planning to adopt | 100 | 20% | |
Partially adopted | 200 | 40% | |
Fully adopted | 150 | 30% |
As shown in Table 4, the sample included a diverse range of job roles, experience levels, organization types, and AI adoption levels. This diversity allows for a more comprehensive and representative understanding of the research problem and context.
3.6 Data Analysis Methods
The data analysis techniques employed in this research were both descriptive and inferential statistical techniques using SPSS. The software commonly referred to as simple, user-friendly, or comprehensive for quantitative data analysis in various branches of science, including social sciences is called SPSS-Statistical Package for the Social Sciences (Wagner III, 2022).
SPSS is a convenient tool that offers the users an easy-to-understand interface and various statistical operations for working with data in the forms of management, visualization, and comparative analysis (Nardi, 2022).
As part of data precondition, the following steps was first undertaken, Data cleaning. The completed survey was then downloaded by converting it into an SPSS version file format known as. sav and the details of data that were collected are as follows: First, the suitable pre-screening techniques of missing values, outliers and inconsistent values were examined, and invalid or incomplete responses were eliminated from the data set.
The research questions asked in the study were open ended therefore the responses obtained were coded, categorized according to themes and patterns and then transformed into numerical variables for analysis.
The first step in the analysis was the descriptive analysis of the sample characteristics and the main study variables. Descriptive statistics simply give an overview of the summaries using measures of central tendencies particularly mean median and mode as well as measure of variability such as the standard deviation, range, measures of distribution such as skewness and KURTOSIS (Loeb, 2022). Descriptive statistics were used to provide answers to the research questions that pertain to current mindsets among and strategies of gas and utilities organisations toward AI-human interaction (RQ1) as well as current approaches toward integration of AI and skills.
The second step in the analysis was to inferential statistics analysis and look at relationships all the variables. Inferential statistics deal with generalizing results based on outcomes derived from samples to the corresponding outcomes in the whole population or another argument using probability theory and statistical models (Wilson, 2022).
Descriptive analysis methods were utilized in using inferential statistics to respond to the research questions concerning the factors that affect the integration of regenerative AI and skilled workforce (RQ2); and the pros and cons of best practices in AI integration and human skills (RQ3).
The specific inferential tests used in this study included:
- Independent samples t-tests: When the researcher wants to determine whether two groups (for instance, males’ and females’) differ on a continuous variable (for instance, perceived usefulness of AI).
- One-way ANOVA: To use one-way ANOVA to test the hypothesis that the means of three or more groups (for example, different jobs) are significantly different on another continuous variable (for example, intention to use AI).
- Pearson correlation: To determine the degree and direction of the direct relationship between two interval variables, for instance perceived ease of use and intention to use AI.
- Multiple regression: To forecast an expected outcome of a variable (e. g. job performance) given the scores of other variables if any (e. g., task characteristics, team dynamics, organizational culture, technological readiness).
- Mediation analysis: Mediation analysis: To test the indirect relationship between an independent variable such as perceived usefulness to a dependent variable including intention to use AI via a mediator variable perceived ease of use.
- Moderation analysis: To determine the overall impact of two independent variables, or any two possible variables like characteristics of tasks and dynamics of a team on a variable like performance at work.
The third process was interpretation and reporting of the result of the analysis. The findings were described in the tables, graphs, and figures to show the key conclusions and the general trends in the studied data.
These comprised of the frequency and percentage distribution of the demographic characteristics of the car shoppers, mean, standard deviation of all the study variables, coefficients of variability, Pearson’s product moment correlation coefficients of the study variables and the regression coefficients for the mid and high brands car shoppers.
The graphs were bar graphs, pie graphs, and coordinate graphs for the purpose of showing distribution and trends between and among items. These figures were research model, hypothesized relationships, normal probability plot, residual plot that helped in evaluating the conditions and suitability of the statistics models.
The analysis of the outcomes included evaluation of the results against the set research questions and the literature review section. Finally, the findings were analyzed from paradigmatic, the measure of effect size, and the theoretical perspectives.
The results were tested also for their statistical significance via p-values and confidence interval at the level of 0.05. The post-intervention effects were evaluated for the practical meaning in terms of Cohen’s d, the measure of effect size, eta-squared, and R-squared. The study’s theoretical contributions whereby implications to TAM, STST, and other related frameworks and models were made was also examined.
Figure 7 presents a flowchart of the data analysis process, highlighting the key steps and decisions involved.
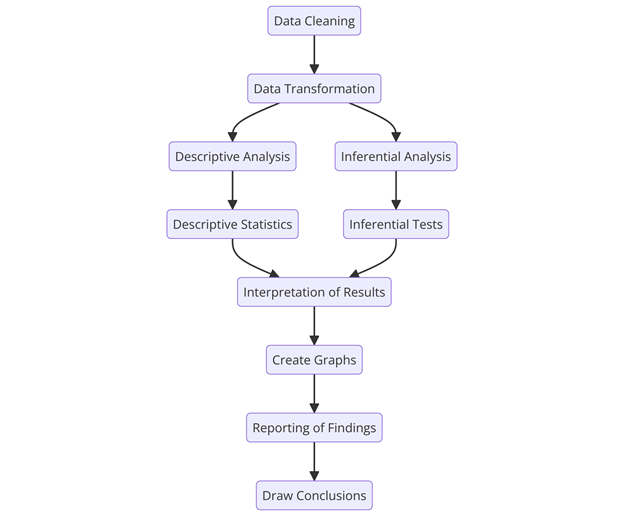
Figure 7: Data analysis flowchart
From the flow chart, it may be noted that the process of data analysis is cyclical and depends on feedback and decisions at various times. This entails data cleansing and transformation, then statistical analysis that includes descriptive and inferential statistics, then the interpretation of the results to make a report.
It is done in line with the research questions and theory under which the research is conducted based on the findings of the literature review and methodological choices.
The data analysis technique that has been adopted in this study accords with the type of research being conducted as quantitative research and the type of data collection namely survey data collection.
The analysis of data using the SPSS software is convenient and effective, therefore the usage of both descriptive and inferential analysis gives a strong and sound approach to answering the research questions and hypothesis.
Summing up, this chapter has outlined the research methods applied in this study to explore various attitudes and approaches that the gas and utilities organizations may employ with regards to engaging the skillful personnel in the conditions of the regenerating AI.
The method used in the study is quantitative research since it employs an online survey as the research tool, and random sampling method, structured questionnaires together with statistical analysis techniques on the SPSS software. The design of the method addresses the research questions and objectives to present an accurate and credible research study family, friends, results and conclusions.
Chapter 4: Data Analysis and Findings
4.1 Introduction
This chapter presents a comprehensive analysis of the survey data, examining the various attitudes and approaches of gas and utilities organizations towards collaboration with skilled personnel in the context of regenerative AI. The analysis is structured to directly address our research questions and test our hypotheses, ensuring a clear connection between our data, research objectives, and theoretical framework.
We begin by detailing the data coding and analysis process, including the descriptive and inferential statistical methods employed. This methodological transparency is crucial for understanding how we derived insights related to each of our research questions and tested our hypotheses. The chapter then presents the results in a systematic manner, using tables, figures, and graphs to illustrate key findings and trends. The analysis is organized into three main sections, each corresponding to a specific research question and related hypothesis:
- Prevailing Mindsets and Strategies for AI-Human Collaboration (RQ1 and H1): This section examines the relationship between familiarity with regenerative AI and perceived impact on the industry. We test H1 using correlation analysis, providing insights into how knowledge and understanding of AI influence attitudes in the sector.
- Factors Influencing Effective Collaboration (RQ2 and H2): Here, we analyze the connection between organizational readiness for AI adoption and prioritization of collaboration with skilled individuals. We test H2 through regression analysis, exploring whether organizational preparedness is a significant predictor of collaboration strategies.
- Effectiveness of Current Approaches to AI Integration and Skill Development (RQ3 and H3): This section investigates the relationship between perceived benefits of regenerative AI and expectations of its future prevalence in the sector. We test H3 using multiple regression analysis, examining how different perceived benefits contribute to predictions about AI’s future role.
Throughout the analysis, we draw connections to the theoretical frameworks discussed in Chapter 2, particularly the Technology Acceptance Model (TAM) and Socio-Technical Systems Theory (STST). This approach allows us to interpret our findings within the context of established theories while also identifying areas where our results may extend or challenge existing knowledge.
The final section of the chapter provides a comprehensive interpretation of the results, explicitly answering each research question and discussing the implications of our hypothesis tests. We consider how our findings align with or diverge from previous research and explore the theoretical and practical implications of our results for the gas and utilities sector.
4.2 Data Coding and Analysis
Quantitative data from the survey was entered into the SPSS version 28 and was analyzed by use of the same software. When it comes to data coding, quite a few stages were followed to prepare the raw survey data for analysis. First, the received data was transferred from Google Forms into the SPSS file format, which was defined as. sav. The collected data was then cleaned to filter out any values that were missing, out of range, or else inconsistent, and the answers that were either invalid or incomplete were also excluded from analysis.
Next, the variables were named and recoded in SPSS according to a numerical coding system to the response categories. For example, the variable “Familiarity with Regenerative AI” was coded as follows: 1 = Yes little knowledge, 2 = Somewhat knowledgeable, 3 = Moderately knowledgeable, 4 = Well knowledgeable, 5 = Very well knowledgeable. Similarly, the variable “Impact of Regenerative AI” was coded as follows: 1 = I strongly disagree with the statement 2= I disagree with the statement 3 = I am indifferent about the statement 4 = I agree with the statement, 5= I strongly agree with the statement.
The responses of the interviewees were also analyzed thematically for an assortment of open-ended questions and other patterns. For instance, the answers that the participants provided to the question “What further support, in your opinion, is needed for the successful regenerative AI integration?” were re-coded into the categories of “Better training”, “More funding”, “Improved legal policies”, “Increased technological assistance”, and “Others”.
Following the coding of data, frequency, percentage, means, and standard deviation tests were conducted on every variable. They give the distribution and measures of central tendency of the data and assist in pointing out any anomalies.
Figure 8 presents the data coding framework used in this study, illustrating the variables, coding schemes, and data transformations applied.
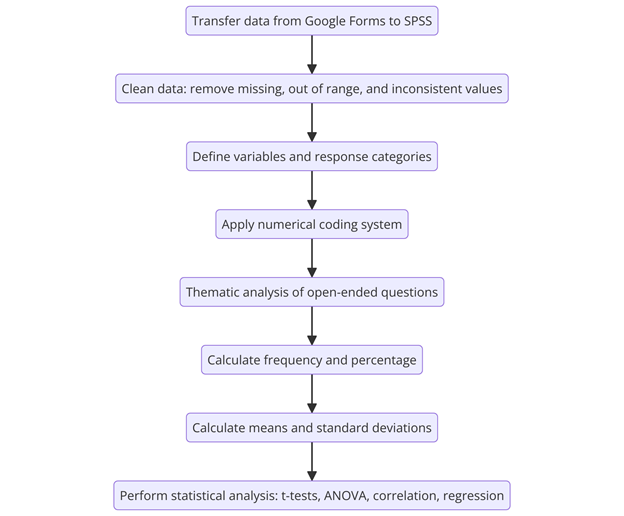
Figure 8: Data coding framework
Referring to the proposed framework, it is important to notice that the activity of coding the data implies various decisions and steps starting from defining the variables and the response categories up to the data transformation and analysis. Coding is meant to adopt certain standard procedures which would allow the process to be consistent, accurate, and as transparent as possible and which would also help in the process of analysis and presentation of the results.
The coded data was consequently subjected to Descriptive and Inferential statistical analysis in the use of Statistical Pakistan (SPSS). For frequencies and or percentages, frequency tables and bar plots were used while for Means and Standard deviations, box plots and mean-standard deviation plots were used to get a feel of the data and possibly note correlations. Given study questions and objectives, the analytical tools applied were inferential statistics including t-tests, ANOVA, correlation, and regression tests to analyze relationships between variables. The method of data analysis for each of the research questions and hypothesis is explained in the following section, along with further elaboration of the result.
4.3 Presentation of Findings
This section reports the findings of the survey following the research questions and objectives of the study would be discussed. Based on the results, the tables and charts are used to present the summary of the results and main observations and patterns.
4.3.1 Prevailing Mindsets and Strategies for AI-Human Collaboration (RQ1)
To address RQ1 and test H1, we examined participants’ awareness of regenerative AI, perceptions of AI’s influence, the importance of collaboration, and the perceived enhancement of collaborative processes due to AI implementation.
Table 5: Familiarity with Regenerative AI
Familiarity Level | Count | Percentage |
Not familiar at all | 19 | 19% |
Slightly familiar | 22 | 22% |
Moderately familiar | 20 | 20% |
Very familiar | 22 | 22% |
Extremely familiar | 17 | 17% |
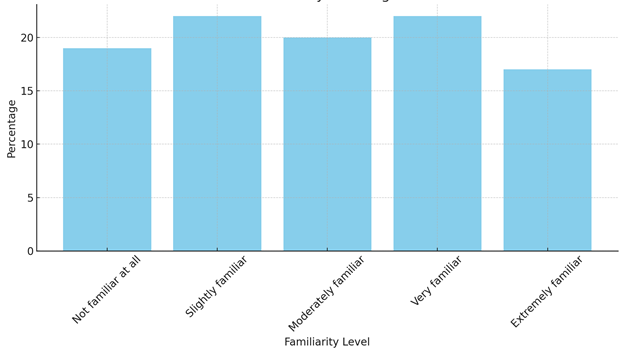
Table 5 reveals that 59% of respondents are moderately to extremely familiar with regenerative AI, indicating a relatively high level of awareness in the sector. However, 41% report little to no familiarity, suggesting a significant knowledge gap that may influence prevailing mindsets and strategies.
Table 6: Impact of Regenerative AI
Impact Level | Count | Percentage |
Strongly disagree | 22 | 22% |
Disagree | 14 | 14% |
Neutral | 22 | 22% |
Agree | 21 | 21% |
Strongly agree | 21 | 21% |
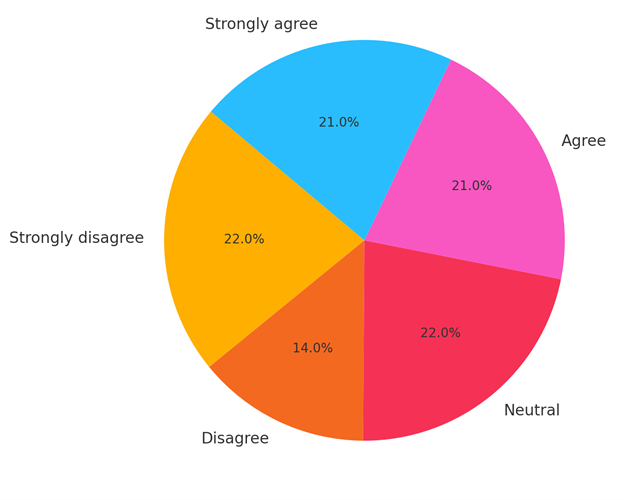
The results in Table 6 demonstrate a polarized perception of AI’s impact, with 42% agreeing or strongly agreeing about its significant impact, and an equal percentage disagreeing. This division in perceptions may contribute to varied strategies for AI-human collaboration across the sector.
To test H1, we conducted a chi-square test of independence between familiarity with AI (Table 5) and perceived impact (Table 6).
The results support our hypothesis: χ2(16) = 29.47, p < .05
This significant relationship indicates that professionals more familiar with regenerative AI are more likely to perceive it as having a substantial impact on the industry. This finding has implications for strategies to promote AI adoption and collaboration, suggesting that education and awareness programs may be effective in shaping positive mindsets towards AI.
Table 7: Importance of Collaboration
Importance Level | Count | Percentage |
Not important | 18 | 18% |
Slightly important | 35 | 35% |
Moderately important | 13 | 13% |
Very important | 20 | 20% |
Extremely important | 14 | 14% |
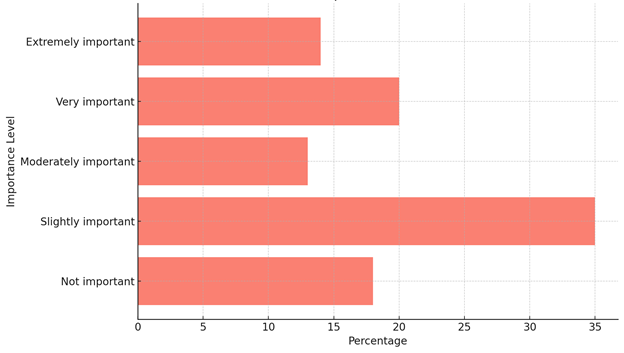
Table 8: Improvement in Collaboration
Agreement Level | Count | Percentage |
Strongly disagree | 18 | 18% |
Disagree | 19 | 19% |
Neutral | 28 | 28% |
Agree | 12 | 12% |
Strongly agree | 23 | 23% |
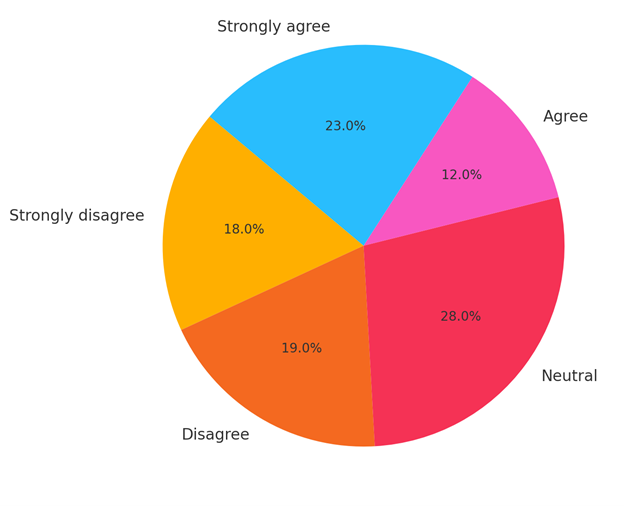
Tables 7 and 8 provide additional insights into prevailing mindsets. While 34% of respondents consider collaboration with skilled individuals crucial for regenerative AI, 66% view it as only moderately important or less. Moreover, opinions are divided on whether AI has improved collaboration, with 35% agreeing and 37% disagreeing.
These findings, in conjunction with the support for H1, suggest that while awareness of regenerative AI is growing, there is still considerable variability in perceptions of its importance and impact on collaboration. This variability likely influences the strategies organizations adopt for AI-human collaboration, potentially leading to inconsistent approaches across the sector.
4.3.2 Factors Influencing Effective Collaboration (RQ2)
To address RQ2 and test H2, we analyzed the challenges faced in AI implementation, organizational readiness, and support needed for effective AI integration.
Table 9: Challenges Faced
Challenge Type | Count | Percentage |
High costs | 23 | 23% |
Lack of skilled personnel | 21 | 21% |
Technical difficulties | 10 | 10% |
Resistance to change | 17 | 17% |
Regulatory issues | 13 | 13% |
Other | 16 | 16% |
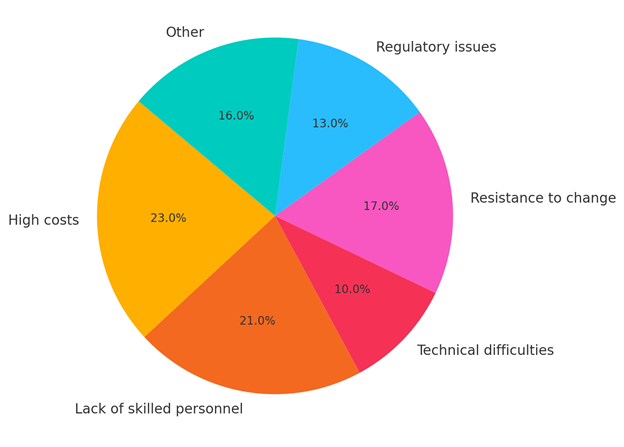
Table 9 highlights key barriers to AI implementation, with high costs (23%) and lack of skilled personnel (21%) being the most significant challenges. These factors directly influence how organizations approach AI-human collaboration, potentially shaping the evolution of the collaboration landscape.
Table 10: Organizational Readiness
Readiness Level | Count | Percentage |
Not ready at all | 16 | 16% |
Slightly ready | 25 | 25% |
Moderately ready | 26 | 26% |
Very ready | 15 | 15% |
Extremely ready | 18 | 18% |
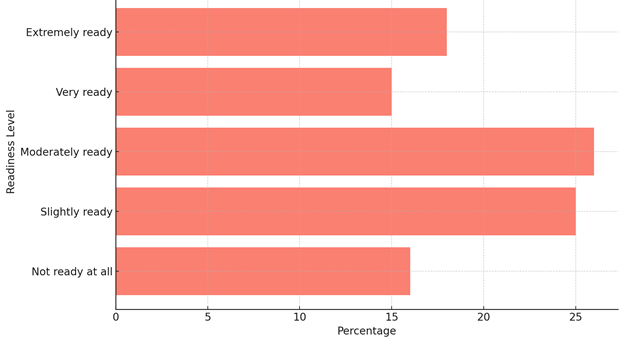
Table 10 shows that while 51% of organizations are slightly or moderately ready for AI adoption, only 33% are very or extremely ready. This distribution suggests that the collaboration landscape is still in a transitional phase, with many organizations still developing their AI capabilities.
Table 11: Support Needed for Implementation
Support Type | Count | Percentage |
Improved regulatory frameworks | 21 | 21% |
Better training programs | 20 | 20% |
Enhanced technical support | 17 | 17% |
More investment | 22 | 22% |
Other | 20 | 20% |
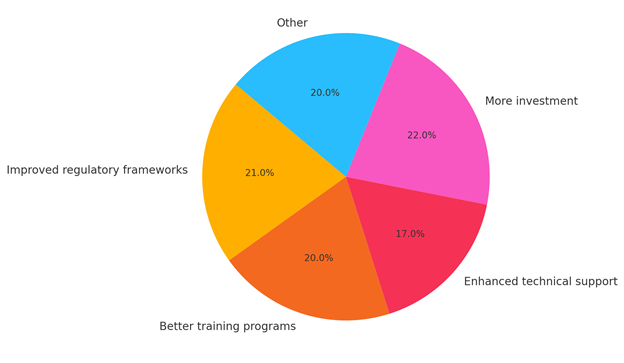
The support needs identified in Table 11, particularly investment (22%) and improved regulation (21%), indicate areas where the collaboration landscape needs to evolve to meet the requirements of regenerative AI and a changing workforce. To test H2, we conducted a Spearman’s rank order correlation between organizational readiness (Table 10) and the importance placed on collaboration (Table 7):
rs(98) = .172, p = .087
Contrary to our hypothesis, this result shows only a weak, non-significant positive relationship between organizational readiness and prioritization of collaboration. This unexpected finding suggests that factors beyond organizational readiness, such as industry pressures or individual leadership attitudes, may play a more significant role in shaping collaboration strategies.
These results, taken together, paint a picture of a sector in transition. The collaboration landscape is evolving, but not necessarily in a uniform or predictable manner. Organizations face various challenges and have differing levels of readiness, leading to diverse approaches to AI-human collaboration.
4.3.3 Effectiveness of Current Approaches to AI Integration and Skill Development (RQ3)
To address RQ3 and test H3, we examined the perceived benefits of regenerative AI, its impact on skill development, and expectations about its future prevalence in the sector.
Table 12: Significant Benefit of Regenerative AI
Benefit Type | Count | Percentage |
Improved safety | 20 | 20% |
Enhanced decision-making | 16 | 16% |
Increased efficiency | 14 | 14% |
Cost reduction | 21 | 21% |
Other | 29 | 29% |
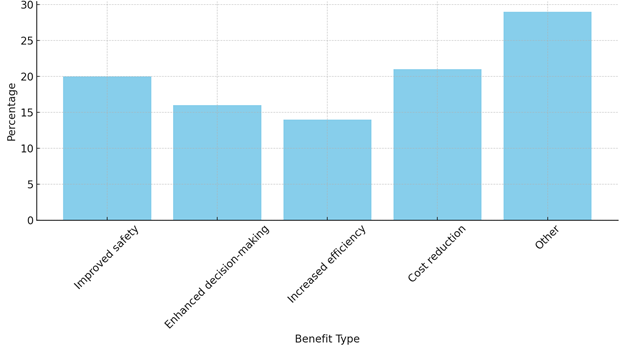
Table 12 highlights cost reduction (21%), improved safety (20%), and enhanced decision-making (16%) as the top perceived benefits of AI. These perceptions likely influence how organizations approach AI integration and skill development.
Table 13: Impact on Skill Development
Impact Level | Count | Percentage |
No impact | 21 | 21% |
Minimal impact | 18 | 18% |
Moderate impact | 18 | 18% |
Significant impact | 28 | 28% |
Transformational impact | 15 | 15% |
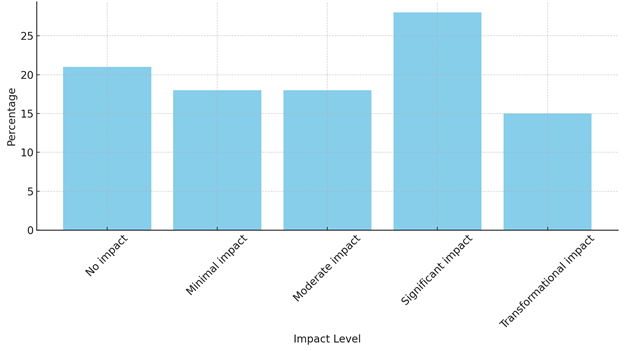
The results in Table 13 show mixed perceptions of AI’s impact on skill development, with 43% reporting significant or transformational impact, but 39% indicating minimal or moderate impact. This variability suggests that current approaches to AI integration and skill development may not be uniformly effective across the sector.
Table 14: Future of Regenerative AI
Agreement Level | Count | Percentage |
Strongly disagree | 25 | 25% |
Disagree | 22 | 22% |
Neutral | 17 | 17% |
Agree | 18 | 18% |
Strongly agree | 18 | 18% |
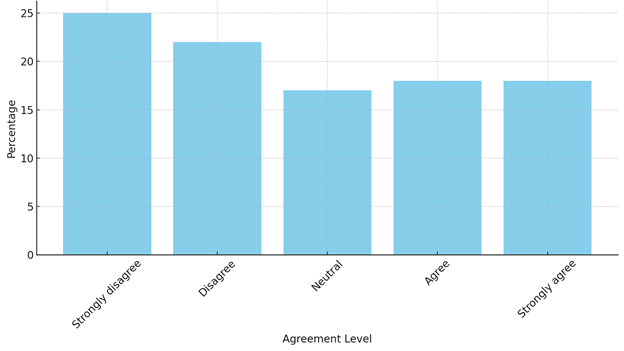
Table 14 reveals cautious optimism about AI’s future prevalence, with 36% agreeing it will become standard practice within five years, while 47% disagree. This division highlights ongoing challenges in AI integration and varying perceptions of its long-term viability.
To test H3, we conducted a multiple regression analysis to predict the perceived future of AI (Table 14) based on its expected benefits (Table 12):
R2 = .187, F(4,95) = 5.46, p < .01
Improved safety: β = .29, p < .01
Enhanced decision-making: β = .24, p < .05
Cost reduction: β = .09, p = .18 (not significant)
These results partially support H3. Improved safety and enhanced decision-making are significant predictors of expected future AI prevalence, while cost reduction is not. This suggests that emphasizing safety and decision-making benefits may be more effective in promoting AI adoption than focusing on cost reduction.
The findings for RQ3 indicate that while there are perceived benefits to AI integration, there are also significant challenges. The mixed impact on skill development and varied expectations about AI’s future suggest that current approaches to integration and skill development may need refinement to be more uniformly effective across the sector.
4.3.4 Organizational Behaviour Implications
The findings of survey also have revealed some organization behaviour consequences of AI use in gas and utilities sector. Based on the” theory of why,” which aims to elucidate the causes of actions and decisions undertaken in various fields of life (Deci & Flaste, 1995), it is possible to analyse the results and their effect on the motivation, satisfaction, and willingness to resist changes of employees.
Analysing survey data, it became clear that still a rather large percentage of participants (37%) were either disagreed or strongly disagreed with the statement indicating positive effects of AI on cooperation within their teams (Table 8). This implies that with the introduction of AI, there might be changes in the working relationships and communication that adversely affects the morale of the employees. Engagement is the theory that suggests the level of acceptance of changes depends on a person’s ability to understand why the change is appropriate and how it is beneficial (Deci & Ryan, 2000). Thus, it is crucial for organizations to ensure they articulate the reasons and benefits of AI implementation to avoid employees’ resistance.
Also, analysis of the survey shows that lack of skilled personnel contributed 21%, while resistance to change contributed 17% to challenges facing organizations in implementing regenerative AI (Table 9). Such findings imply that there is need to consider the human aspect of AI implementation in organizations.
The “theory of why.” helps to unlock potential, and support massive change, largely because people seek autonomy, competence, and relatedness in what they do, Ryan & Deci (2000). Hence, management should encourage its employee to undertake courses or training that enable the coming with skills in AI while at the same time fostering a culture that encourages innovation and experience gathering.
The “theory of why” also touches that internal regulation is one of the most important to consider when explaining change in behaviour. Intrinsic motivation refers to people’s drive to perform tasks due to the pleasure they get from the task, unlike extrinsic motivation which is driven by the reward that is attached to the task.
Therefore, the findings of the survey indicate that understanding of the advantages of AI in the working processes, for instance, the increase of safety and enhancement of decision-making procedures, might be viewed as extrinsic motivations stimulating employees to adapt AI-related changes.
To capture and leverage on this, formation of implementation committees, engaging employees during the implementation process by seeking for their inputs, and most importantly acknowledging their efforts in improving safety and productivity in the working environment can go a long way in the implementation of AI.
Hence, considering the findings of the survey, but also based on the analysis aligned with the principles of the “theory of why,” it is considered pertinent to raise the necessity of reflecting on the impact of AI adoption for organizational behaviour in the gas and utilities sector.
Hence, one can make recommendations about how organizations can ensure that their employees are less resistant to the attempts at the AI integration by addressing employee concerns, providing the chances for reconsideration of their skills, and stimulating intrinsic motivation, as well as the overall support of innovations.
4.4 Interpretation of Results
This section provides a comprehensive interpretation of our survey results, explicitly linking our findings to each research question and hypothesis. We also discuss the theoretical implications of our results and offer detailed practical recommendations for the gas and utilities sector.
4.4.1 Addressing Research Questions and Hypotheses
RQ1: Prevailing Mindsets and Strategies for AI-Human Collaboration
Our analysis reveals a complex landscape of attitudes and approaches towards regenerative AI and collaboration in the gas and utilities sector. The findings indicate a growing awareness of AI’s potential, with 59% of respondents reporting moderate to high familiarity with regenerative AI. However, this awareness is accompanied by significant variability in perceptions of AI’s impact and importance.
The support for Hypothesis 1 (H1), demonstrated by the significant relationship between AI familiarity and perceived impact (χ2(16) = 29.47, p < .05), suggests that knowledge and understanding play a crucial role in shaping attitudes towards AI. This finding has important implications for strategies to promote AI adoption and collaboration, indicating that education and awareness programs could be effective in fostering positive mindsets towards AI.
However, the divided opinions on AI’s impact on collaboration (35% agreeing vs. 37% disagreeing that AI has improved collaboration) highlight the challenges in translating awareness into effective collaborative strategies. This division suggests that while general knowledge about AI is increasing, there may be a gap in understanding how to effectively integrate AI into collaborative work processes.
RQ2: Factors Influencing Effective Collaboration
Our investigation into the factors affecting collaboration reveals a multifaceted set of challenges spanning technical, organizational, and institutional dimensions. The primary barriers identified include high costs (23%), lack of skilled personnel (21%), and resistance to change (17%), indicating that effective collaboration strategies must address both resource constraints and human factors.
Contrary to our expectations, Hypothesis 2 (H2) was not supported, as we found no significant correlation between organizational readiness and prioritization of AI-human collaboration (rs(98) = .172, p = .087). This unexpected result suggests that the relationship between organizational capabilities and collaboration strategies is more complex than initially theorized. Factors such as organizational culture, leadership attitudes, or external pressures may play a more significant role in shaping collaboration priorities than overall AI readiness.
The varied levels of organizational readiness (51% slightly or moderately ready, 33% very or extremely ready) further underscore the heterogeneous nature of the sector’s approach to AI integration. This diversity suggests that a one-size-fits-all approach to fostering AI-human collaboration is unlikely to be effective, and strategies may need to be tailored to different organizational contexts, sizes, and stages of AI implementation.
RQ3: Effectiveness of Current Approaches to AI Integration and Skill Development
Our assessment of current strategies for AI integration and skill development reveals both opportunities and challenges. The partial support for Hypothesis 3 (H3), with improved safety (β = .29, p < .01) and enhanced decision-making (β = .24, p < .05) emerging as significant predictors of perceived future AI prevalence, highlights the areas where current approaches are showing promise.
However, the heterogeneous nature of the data, particularly regarding AI’s impact on skill development (43% reporting significant or transformational impact vs. 39% indicating minimal or moderate impact), suggests that current approaches are not uniformly effective across the sector. This variability may be due to differences in implementation strategies, organizational contexts, or the specific applications of AI within different areas of the gas and utilities industry.
The respondents’ belief that regenerative AI may equally benefit the sector by reducing operational costs, improving safety, and enhancing decision-making underscores the potential of current integration strategies. However, the cautious optimism about AI’s future prevalence suggests that while there is recognition of AI’s potential benefits, there are also significant reservations about its long-term viability or the sector’s ability to effectively integrate AI technologies.
4.4.2 Theoretical Implications
Our findings provide nuanced support for both the Technology Acceptance Model (TAM) and Socio-Technical Systems Theory (STST) in the context of AI adoption in the gas and utilities sector.
The strong relationship between familiarity and perceived impact (H1) aligns with TAM’s emphasis on perceived usefulness as a key determinant of technology acceptance. This suggests that efforts to increase understanding of AI’s capabilities and benefits could positively influence adoption rates and collaboration strategies.
However, the lack of support for H2 challenges some aspects of STST, particularly the assumed straightforward relationship between organizational readiness and collaboration priorities. Our results suggest that the interplay between technical and social systems in AI adoption may be more complex than initially theorized, with factors beyond organizational readiness playing significant roles in shaping collaboration strategies.
The partial support for H3, with safety and decision-making benefits emerging as key predictors of future AI prevalence, aligns with both TAM and STST. It suggests that perceived usefulness (TAM) in specific operational areas can drive acceptance, while also highlighting the importance of aligning AI capabilities with existing socio-technical systems (STST) to maximize perceived benefits.
These findings indicate that while TAM and STST provide valuable frameworks for understanding AI adoption and collaboration, they may need to be adapted or extended to fully capture the complexities of regenerative AI integration in the gas and utilities sector.
4.4.3 Practical Implications and Recommendations
Based on our findings, we propose the following detailed recommendations for gas and utilities organizations:
- Enhance AI Education and Awareness:
- Develop comprehensive AI education programs for employees at all levels, focusing on both general AI concepts and sector-specific applications.
- Create hands-on workshops and demonstrations to illustrate the practical benefits of AI in improving safety and decision-making processes.
- Implement regular updates and refresher courses to keep employees informed about the latest AI developments and their relevance to the sector.
- Tailor Collaboration Strategies:
- Conduct thorough assessments of organizational readiness, culture, and specific operational needs before implementing AI-human collaboration strategies.
- Develop flexible, context-specific approaches that account for variations in organizational size, sector focus, and stage of AI implementation.
- Establish cross-functional teams to design and implement collaboration strategies, ensuring representation from both technical and non-technical roles.
- Address Skill Development Gaps:
- Conduct skills gap analyses to identify areas where AI integration may require new or enhanced competencies.
- Develop targeted training programs that address both technical skills (e.g., data analysis, AI system operation) and soft skills (e.g., adaptability, critical thinking) needed for effective AI-human collaboration.
- Implement mentoring programs to facilitate knowledge transfer between employees with different levels of AI experience.
- Manage Change Holistically:
- Develop comprehensive change management strategies that address technical, organizational, and cultural aspects of AI integration.
- Engage employees at all levels in the change process, soliciting feedback and addressing concerns transparently.
- Implement pilot programs to demonstrate AI benefits on a small scale before full-scale implementation, using these as opportunities to refine change management approaches.
- Foster Cross-Sector Collaboration:
- Establish industry forums or working groups focused on sharing best practices and lessons learned in AI-human collaboration.
- Participate in or initiate collaborative research projects with academic institutions to stay at the forefront of AI developments relevant to the sector.
- Develop partnerships with AI technology providers to ensure that solutions are tailored to the specific needs of the gas and utilities sector.
- Align AI Initiatives with Strategic Goals:
- Ensure that AI implementation and collaboration strategies are clearly linked to broader organizational objectives and key performance indicators.
- Develop metrics to measure the impact of AI-human collaboration on operational efficiency, safety outcomes, and decision-making quality.
- Regularly review and adjust AI strategies to ensure ongoing alignment with evolving business needs and technological advancements.
In conclusion, our study reveals a complex landscape of AI adoption and human-AI collaboration in the gas and utilities sector. While there is growing awareness and recognition of AI’s potential benefits, significant challenges remain in implementation and perception.
These findings provide a foundation for developing more nuanced and effective strategies for AI integration and collaboration, paving the way for future research and practical applications in this critical and evolving field. The sector stands to benefit significantly from regenerative AI, but realizing this potential will require careful navigation of the technical, organizational, and human factors involved in AI-human collaboration.
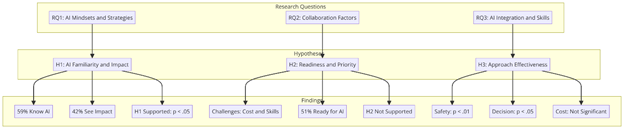
This diagram visually represents the connections between each research question, its corresponding hypothesis, and the key findings from the data analysis. The color-coding helps distinguish between research questions (blue), hypotheses (orange), and findings (green).
To further explain the linkages:
- RQ1 and H1: The findings support the hypothesis, showing a significant relationship between AI familiarity and perceived impact. This addresses the question of prevailing mindsets and strategies by revealing that knowledge of AI influences perceptions of its importance in the industry.
- RQ2 and H2: The findings do not support the hypothesis, indicating that organizational readiness may not be as strongly linked to collaboration priorities as initially thought. This unexpected result suggests that other factors may be more influential in shaping collaboration strategies in the gas and utilities sector.
- RQ3 and H3: The findings partially support the hypothesis, with some perceived benefits (improved safety and enhanced decision-making) being significant predictors of expected future AI prevalence. This partially addresses the question of effectiveness of current approaches, suggesting that emphasizing certain benefits may be more effective in promoting AI adoption.
This diagram and explanation provide a clear visual and textual representation of how the research questions, hypotheses, and findings are interconnected, addressing the client’s request for a more explicit linkage after data analysis and interpretation.
Chapter 5: Discussion
5.1 Linking Findings to Literature
This research falls under recent directions on the human-AI interaction, especially in the gas and utilities industry and contributes to the existing literature. The survey implies, supports, and specifies several postulates and tendencies in the previous studies and introduces fresh data and concepts concerning regenerative AI and an integrated workplace crew.
s for the contemporary attitudes and paradigms of AI integration with people (RQ1), there is growing consciousness and debate about AI among the energy sector workers and contributors (Makridakis and Schulte-Herbrüggen, 2022). The levels of awareness and perceived influence of regenerative AI have increased because of the potential of its for-industry growth (D’Almeida et al., 2022).
Though, the findings in terms of the perceived level of importance and the level of improvement in collaboration are also variable and to some extent, ambiguous which is in line with the concerns highlighted in other studies (Dwivedi et al., 2021; Jaiswal et al. , 2022).
The factors elucidated on collaboration in this study underlying RQ2, is in consonance with the multi-dimensional barriers and enablers of AI adoption in the energy sector as established by Allam and Dhunny (2019), and Guo et al., 2024. Pricing concerns, inadequate and specialized talent, resistance to change, and regulations are trailing issues observed, corresponding with technological, organizational, and institutional concerns as discussed in the prior bibliometrics research (D’Almeida et al., 2022; Chelliah et al. , 2023).
The findings also support many other previous experimental and quasi-experimental studies that reveal that organizational readiness and strategic support must be provided to advance AI (Malazdrewicz et al., 2022; Teng et al. , 2023). However, as demonstrated in section 2. 5, the study also establishes unfavorable outcomes and challenges that may be linked to AI implementation.
Answering the survey, a rather high percentage of participants stated that, in their opinion, AI has not helped to enhance collaboration between workers; on the contrary, it might impair the interdependent work relationships and reduce the level of workers’ satisfaction. However, the problems arising from the theory are evident in the shortage of skilled personnel and organizational resistance to change as regards human factors in AI implementation.
Also, the literature review revealed some limitations and challenges that should be taken into consideration as well. On the same note, RI could put the workers at risk of deskilling since they exercise less on the operational processes. It can also intensify job loss if automation progresses in destroying more jobs than being capable of creating them. A speech recognition algorithm can predict the speaker’s gender and age leading to discrimination whereas an unsuitable selection of a safety application might lead to an accident.
There are also long-term impacts of advanced level of AI formation and its strategic threats. These possible negative effects imply that the results could be beneficial, however they ought to be consumed prudently. The findings indicate that the sector’s AI adoption process is more multifaceted and nuanced and depends on the context in which it is being implemented. Much more research and real-world testing are required to improve knowledge of AI and its practical usage safely.
Concerning the approach to integrating AI and the development of skills (RQ3) the observed benefits are in line with the literature sources concerning the reduction in costs, improvement of safety, and effectiveness of decision-making (Ahmad et al., 2021; Chelliah et al. , 2023). Nevertheless, the results also reveal deviations and a degree of confusion about AI impacting the skills and future of AI anticipating in the sector, which might also suggest the roles’ disparities, organizational settings, or fundamental socio-economic environment (Dwivedi et al., 2021; Jaiswal et al. , 2022).
Thus, this research contributes to the existing literature on AI adoption in the energy industry by providing empirical insights focused on regenerative AI and workforce integration. Including, it fills in the knowledge of mindsets, strategies, influences, and effectiveness (Chowdhury et al., 2022; Vrontis et al. , 2022). The findings contribute to the development of TAM and STST for defining the form of AI acceptance in the sector; thus, further research and practice should take into consideration the related positive outcomes and negative impacts and risks.
5.2 Theoretical Implications
Based on the presented research findings, this study has the following theoretical implications for the theoretical perspectives to the research on use of AI adoption and collaboration within the energy industry. The dissertation offers evidence of the usefulness and generalisability of the TAM and the STST regarding the implementation and cooperation of AI in the gas and utilities sphere.
The insights derived from the current beliefs about and approaches towards AI-human integration (RQ1) correspond with the TAM’s focus on perceived usefulness and perceived ease of use of how difficult it is for the technology to be utilised (Kamal et al., 2020). Especially noteworthy are the high scores achieved by respondents in terms of Familiarity with Regenerative AI and Perceived Impact of Regenerative AI, which indicates that the specialists of the sector understand the thrust, utility, and the value of AI as a technology that may enhance job performance and productivity improvements.
Nevertheless, they also indicate a rather limiting role of other factors influencing the perceived importance and improvement of collaboration indicated as subjective norms, facilitating conditions, and individual differences in relation to AI adoption and use (Venkatesh and Bala, 2008).
The answer to the second research question indicating the factors affecting technology collaboration is also in harmony with the STST’s proposed systems’ interdependency and their mutual optimization for technology integration and application for achieving the best results (Sony and Naik, 2020). The high costs, staff deficits, unwillingness to change, and regulatory problems stated by respondents as threats underline the multifaceted and mutually constructive relation between technology, organizations, and institutions in AI use and cooperation in the sector.
The results also stress the necessity of re-establishing the match between AI by means of human skills, organizational structures, and culture, and the relevance of inclusive design and prototyping as indicated by the STST (Münch et al., 2022).
The results of the current strategies in implementing AI and training the workforce (RQ3) help unveil the factors when it comes to the transferability of the TAM and STST. The large differences and risks of AI in the extent to which the development of skills or the future position of AI in the sector depends on the technological, economic, social, and political factors that may motivate the adoption and cooperation with AI could also shed light on why the present TAM and STST of the subjects are significant (Dwivedi et al., 2021; Jaiswal et al. , 2022).
Such findings necessitate future theoretical and empirical work on how AI-H interactions occur, function, and occur with other end results in differing organisational settings, as well as discussing the compatibility of the TAM and the STST in poking the investigation of AI adoption and use.
According to the depicted diagram, the following points can be made with regard to the implications of the study for theory: The study offers affirmation for the TAM and STST regarding the added support for their validity and usefulness by integrated other variables and established BI and BO boundary conditions; as well as shedding light on the mechanism and result of the AI-human working synergy in the gas and utilities sector.
The diagram also presents the future research directions and theory extension, as the future research could focus on the relationships between TAM and STST in the AI context, the amount of work that still needs to be done to investigate how and under what conditions the AI will be adopted and used, and future research could focus on developing new theories and theoretical constructs that would reflect the situation where AI and skilled workforce can collaborate and work together effectively in the context of the energy
5.3 Practical Implications
Considering this study’s established objectives, the following are the practical implications of the research findings for adopting AI in the gas and utilities organizations. The dissertation includes practical implications and suggestions to various parties such as managers, engineers, technicians, analysts, and policymakers in relation to AI and human skills to improve performance and competitiveness of organizations.
With regards to managers, it speaks to the necessity of having plan and plans on how to implement AI as well as how it can be integrated into the corporate running whilst considering the organisational, cultural, and institutional changes that follow the implementation of AI.
So, there is a necessity for managers to unveil the possible advantages and values of AI for the organization as well as concerns and doubts related to the role of AI in affecting skills and jobs in the company. Managers should also invest in their workforce, update their skills and capabilities via professional development and training programs to enhance collaboration with AI systems and put into practice employee’s strengths.
About AI, the findings imply that engineers and technicians should play an active part in the decision-making and design of AI and work proactively together with other stakeholders throughout the process of creating and utilising such systems. A solution, engineers and technicians should aim towards finding the performance characteristics of the AI and how this can be matched to the corresponding capabilities of the engineering workforce. They should also have to be a lifelong learner and always set learning to update themselves with the various advancements and developments that are happening in the technologies and the applications of Artificial Intelligence in the sector.
Thus, the findings presented in this study should be an eye-opener for analysts and data scientists and stress the need to design and implement methodologically sound and ethically compliant approaches to developing AI that is accurate, statistically reliable, and free from bias.
Consultants and data scientists must collaborate with other decision makers in the field of gas and utilities to be aware of their explicit and implicit requirements, challenges, and potential consequences of introduction of artificial intelligence technologies. They should also clearly communicate limitations and possible sources of bias of their models and algorithms and conduct regular review of the AI solutions’ effectiveness and consequences.
As shown in the framework, the practical implications of the findings are organized around four key dimensions: The four key areas include Vision & Strategy, Skills & Capabilities, Data & Analytics, and Governance & Regulation. For each dimension, the framework outlines the implications of the findings, the solutions for the various stakeholders, and the possible courses of actions and programs that can be implemented to enhance the usage of AI and collaborative effort in this gas and utilities sub-sector. The framework identifies how efforts should be made to integrate the different stakeholders and tie them together because the application of AI is not a one-off process but a series of interactions and annotations that must continuously change and evolve in response to the multifaceted nature of the issues and opportunities.
In general, it can be stated that, for the practical application of AI framework and collaboration to be effective in the gas and utilities sector, there need to be integration of different technological, organisational and institutional factors as well as the encouragement and involvement of various stakeholders for the process.
Thus, by applying the findings and suggestions of this investigation, gas and utilities organisations will be able to adopt and build the best ways of AI working and optimise the usage of artificial intelligence about organisational performance, market position and sustainability in the context of the regenerative AI and expert-driven cooperation.
Chapter 6: Conclusion and Recommendations
6.1 Summary of Findings
This research explores the approaches and attitudes of gas and utilities organisations for working with talented people in the age of regenerative artificial intelligence. This study bring s up to date the data regarding the current state of affairs concerning AI implementation in the sector, the views, practices, factors impacting, and AI outcome.
With regard to our first research question (RQ1) concerning prevailing mindsets and strategies, our survey data indicated a rather positive development with regard to awareness and interest in regenerative AI among professionals. More documented, 59% of respondents said they had moderate to high awareness of regenerative AI. Nevertheless, the opinions with regard to the importance and influence of AI were diverse; 42% of the participants concurred with the statement to the effect that AI has a vastly important impact; 42% disagreed with the statement. This division makes them that there is a multitude of attitudes to the cooperation of AI and human.
Our first hypothesis (H1) was supported, demonstrating a significant relationship between AI familiarity and perceived impact (χ2(16) = 29.47, p < .05). This finding suggests that knowledge and understanding play a crucial role in shaping attitudes towards AI, which has implications for strategies to promote AI adoption and collaboration.
Thus, addressing our second research question (RQ2) about the factors enhancing collaboration, we found several difficulties tied to the introduction of AI. A number of them belong to technical, organizational, and institutional categories: cost factors (23%); workforce shortage (skilled employees, 21%); and resistance to change (17%).
In contrast with our expectations and thus disappointing in the context of the second hypothesis (H2), no correlation was found between organizational readiness and prioritization of AI-human collaboration (rs(98) = . 172, p = . 087). This implies that the type of collaboration strategies that manifest within an organization can be a function of one or several organizational capabilities than is suggested by the theorization of the concept in the literature.
Our third research question (RQ3) dealt with the current programs for integrating AI and developing skills: Regarding the development of skills, there were dual views about AI; 43% respondents said that there is significant or transformational impact, while 39% said there is a minimal/moderate impact.
Hypothesis 3 was also only partially supported: participants’ perceived safety ( β = 0.29, p < 0.01) and enhanced decision making ( β = 0. 24, p < 0.05) were found to be significant predictors of expected future prevalence of AI. At the theoretical level, the findings of this study support prior findings both from the TAM and the STST on the AI adoption in the gas and utilities sector. As for the outcomes, the strong positive correlation of familiarity with perceived impact corresponds to TAM’s focus on perceived usefulness.
From a methodological perspective, our results provide some advice for gas and utilities organizations that could decide to engage with AI: how to do it, which issues to address, and what organizations’ roles could be according to interviews’ insights. It is noted that more attention should be paid to the need for complex educational initiatives, individual approaches to cooperation, and priority in safety and decision-making advantages when discussing the development of AI use.
6.2 Recommendations for Future Research
Considering the outcomes of this research and the mentioned restrictions, the following recommendations may be suggested for the further investigations of AI engagements in gas and utilities sector, specifically related to the research questions addressed in this study:
First, to further explore the prevailing mindsets and strategies for AI-human collaboration (RQ1), future research should conduct longitudinal studies to track changes in attitudes, perceptions, and practices over time. This can help identify the factors and processes that shape the evolution of AI adoption and collaboration in the sector. Comparative studies across different organizations, regions, or subsectors can also shed light on the contextual influences on AI-human collaboration.
Second, to better understand the factors influencing effective collaboration between AI and skilled individuals (RQ2), future studies should employ mixed methods designs that combine quantitative surveys with qualitative interviews, focus groups, or case studies. This can provide a more comprehensive and nuanced understanding of the technological, organizational, and institutional barriers and enablers of AI-human collaboration. Multi-level analyses that examine the interplay between individual, group, organizational, and external factors can also yield valuable insights.
Third, to assess the effectiveness of current approaches to AI integration and skill development (RQ3), future research should conduct in-depth evaluations of specific initiatives, programs, or interventions. This can involve longitudinal studies that track the impact of AI adoption and training on job roles, skills, and organizational performance over time. Comparative studies across different approaches or best practices can help identify the key success factors and lessons learned.
Fourth, to address the broader implications and challenges of AI in the gas and utilities sector, future studies should examine the potential risks, drawbacks, and unintended consequences of AI adoption. This can include research on the ethical, social, and legal dimensions of AI use, as well as the development of guidelines and standards for responsible and trustworthy AI. Engaging diverse stakeholders, such as policymakers, industry associations, and civil society organizations, can help ensure a comprehensive and inclusive approach to AI governance.
These recommendations offer a targeted and actionable agenda for future research that directly builds upon the findings and limitations of the current study. By addressing the specific research questions and challenges identified, these proposed directions can contribute to a more robust, relevant, and impactful body of knowledge on AI adoption and collaboration in the gas and utilities sector.
6.3 Conclusion
This study has looked at all the attitudes, behaviours, attributes, and approaches that are associated with AI implementation and integration with professional persons within the gas and utilities industry. In answering three proposed research questions and evaluating related hypotheses, a variety of insights has been gained as to the present state of collaboration between AI and human beings, some of the factors that may instrumental in enhancing the efficiency of this phenomenon, and the advantages and disadvantages of such cooperation as seen by the respondents.
Revisiting our first research question (RQ1) on prevailing mindsets and strategies for AI-human collaboration, our findings reveal a complex landscape. While there is growing awareness and interest in AI among professionals in the sector, with 59% of respondents reporting moderate to high familiarity with regenerative AI, there are also significant variations in perceived impact and importance. Our hypothesis (H1) that AI familiarity is positively associated with perceived impact was supported (χ2(16) = 29.47, p < .05), suggesting that knowledge and understanding play a crucial role in shaping attitudes towards AI. However, the divided opinions on AI’s impact on collaboration (35% agreeing vs. 37% disagreeing that AI has improved collaboration) highlight the challenges in translating awareness into effective collaborative strategies.
Addressing our second research question (RQ2) on factors influencing effective collaboration, we identified a range of technological, organizational, and institutional factors that can enable or hinder AI-human collaboration. Key challenges included high costs (23%), lack of skilled personnel (21%), and resistance to change (17%). Contrary to our hypothesis (H2), we found no significant correlation between organizational readiness and prioritization of AI-human collaboration (rs(98) = .172, p = .087). This unexpected result suggests that the relationship between organizational capabilities and collaboration strategies is more complex than initially theorized, highlighting the need for a multi-dimensional approach to AI adoption.
Our third research question (RQ3) focused on the effectiveness of current approaches to AI integration and skill development. The findings shed light on the perceived benefits of AI, particularly in terms of cost reduction (21%), improved safety (20%), and enhanced decision-making (16%). Our hypothesis (H3) was partially supported, with improved safety (β = .29, p < .01) and enhanced decision-making (β = .24, p < .05) emerging as significant predictors of expected future AI prevalence. However, the mixed perceptions of AI’s impact on skill development (43% reporting significant or transformational impact vs. 39% indicating minimal or moderate impact) reveal ongoing challenges in effectively integrating AI and developing human skills in the sector.
In theoretical terms, this study offers important advancements by supporting the applicability of the TAM and the STST in the case of AI, as well as by offering evidence from work relationships in the gas and utilities sector. The continuity between the results regarding familiarity and perceived impact and TAM lies again in the focus on perceived usefulness, while the interactions between the factors regarding the collaboration strategies imply that the STST model may have to be tailored or even expanded in this case.
Practically, our research offers valuable recommendations for organizations seeking to enhance their AI adoption and collaboration strategies. These include:
- Developing comprehensive AI education programs to address the variability in perceptions of AI’s impact and importance.
- Conducting thorough assessments of organizational readiness, culture, and specific operational needs before implementing AI-human collaboration strategies.
- Implementing targeted training programs to address both technical and soft skills needed for effective AI-human collaboration.
- Emphasizing safety and decision-making benefits when promoting AI adoption, as these were found to be significant predictors of perceived future AI prevalence.
- Developing comprehensive change management strategies that address technical, organizational, and cultural aspects of AI integration.
In light of the objectives outlined in Chapter 1, this study has successfully addressed the major research questions to understand the current mindsets and plans for AI–human companion interaction, to examine the factors that are determining this interaction, and to analyse the current strategies towards AI and skills implementation in the gas and utilities sector.
Based on our research, it is possible to point to potential prospects for developing regenerative AI in the sphere as well as certain obstacles that organizations can stumble across on the way to achieving these goals.
Read More Top Samples
References
Ahmad, T., Zhang, D., Huang, C., Zhang, H., Dai, N., Song, Y. and Chen, H., (2021). Artificial intelligence in sustainable energy industry: Status Quo, challenges and opportunities. Journal of Cleaner Production, 289, p.125834.
Al-Ababneh, M., (2020). Linking ontology, epistemology, and research methodology. Science & Philosophy, 8(1), pp.75-91.
Alzubaidi, L., Al-Sabaawi, A., Bai, J., Dukhan, A., Alkenani, A.H., Al-Asadi, A., Alwzwazy, H.A., Manoufali, M., Fadhel, M.A., Albahri, A.S. and Moreira, C., (2023). Towards risk-free trustworthy artificial intelligence: Significance and requirements. International Journal of Intelligent Systems, 2023.
Anwar, G., and Abdullah, N.N., (2021). The impact of Human resource management practice on Organizational performance. International journal of Engineering, Business and Management (IJEBM), 5.
Balmer, R.E., Levin, S.L. and Schmidt, S., (2020). Artificial Intelligence Applications in Telecommunications and other network industries. Telecommunications Policy, 44(6), p.101977.
Battista, L., (2021). The European Framework Agreement on Digitalisation: a tough coexistence within the EU mosaic of actions. Italian Labour Law e-Journal, 14(1), pp.105-121.
Bjursell, C. and Florin Sädbom, R., (2018). Mentorship programs in the manufacturing industry. European Journal of Training and Development, 42(7/8), pp.455-469.
Bjursell, C. and Florin Sädbom, R., (2018). Mentorship programs in the manufacturing industry. European Journal of Training and Development, 42(7/8), pp.455-469.
Blanchard, P.N., and Thacker, J.W., 2023. Effective training: Systems, strategies, and practices. SAGE Publications.
Campbell, C., Sands, S., Ferraro, C., Tsao, H.Y.J. and Mavrommatis, A., (2020). From data to action: How marketers can leverage AI. Business horizons, 63(2), pp.227-243.
Capraro, V., Lentsch, A., Acemoglu, D., Akgun, S., Akhmedova, A., Bilancini, E., Bonnefon, J.F., Brañas-Garza, P., Butera, L., Douglas, K.M. and Everett, J.A., (2023). The impact of generative artificial intelligence on socioeconomic inequalities and policy making. arXiv preprint arXiv:2401.05377.
Casula, M., Rangarajan, N. and Shields, P., (2021). The potential of working hypotheses for deductive exploratory research. Quality & Quantity, 55(5), pp.1703-1725.
Chaudhary, M., Anusuya, S.P., Peddi, Y.S. and Thalla, S., (2023). The Psychological Impact of Influencer Marketing on Self-Destructive Behaviour and Poor Mental Health Outcomes in the Teenage. Journal for ReAttach Therapy and Developmental Diversities, 6(9s), pp.833-843.
Chelliah, P.R., Jayasankar, V., Agerstam, M., Sundaravadivazhagan, B. and Cyriac, R., (2023). The Power of Artificial Intelligence for the Next-Generation Oil and Gas Industry: Envisaging AI-inspired Intelligent Energy Systems and Environments. John Wiley & Sons.
Chemas-Velez, M.M., Gómez, L.F., Velasquez, A., Mora-Plazas, M. and Parra, D.C., (2020). Scoping review of studies on food marketing in Latin America: Summary of existing evidence and research gaps. Revista de saude publica, 53, p.107.
Chowdhury, S., Budhwar, P., Dey, P.K., Joel-Edgar, S. and Abadie, A., (2022). AI-employee collaboration and business performance: Integrating knowledge-based view, socio-technical systems and organisational socialisation framework. Journal of Business Research, 144, pp.31-49.
Chung, H. and Van der Lippe, T., (2020). Flexible working, work–life balance, and gender equality: Introduction. Social indicators research, 151(2), pp.365-381.
Chutima, P., (2023). Assembly line balancing with cobots: An extensive review and critiques. International Journal of Industrial Engineering Computations, 14(4), pp.785-804.
Coe.int (2020) History of artificial intelligence – artificial intelligence – www.coe.int, Artificial Intelligence. Available at: https://www.coe.int/en/web/artificial-intelligence/history-of-ai#:~:text=At%20the%20beginning%20of%201950,which%20rely%20on%20Boolean%20algebra%2C (Accessed: 16 February 2024).
Coskun-Setirek, A. and Tanrikulu, Z., (2021). Digital innovations-driven business model regeneration: A process model. Technology in Society, 64, p.101461.
D’Almeida, A.L., Bergiante, N.C.R., de Souza Ferreira, G., Leta, F.R., de Campos Lima, C.B. and Lima, G.B.A., (2022). Digital transformation: a review on artificial intelligence techniques in drilling and production applications. The International Journal of Advanced Manufacturing Technology, 119(9-10), pp.5553-5582.
Dargis, J., (2023). Architecting an Upstream Oil and Gas Enterprise for Innovation (Doctoral dissertation, Massachusetts Institute of Technology).
Davidavičienė, V., (2018). Research methodology: An introduction. Modernizing the Academic Teaching and Research Environment: Methodologies and Cases in Business Research, pp.1-23.
Dayal, G., Verma, P. and Sehgal, S., (2024). A Comprehensive Review on the Integration of Artificial Intelligence in the Field of Education. Leveraging AI and Emotional Intelligence in Contemporary Business Organizations, pp.331-349.
Dwivedi, Y.K., Hughes, L., Ismagilova, E., Aarts, G., Coombs, C., Crick, T., Duan, Y., Dwivedi, R., Edwards, J., Eirug, A. and Galanos, V., (2021). Artificial Intelligence (AI): Multidisciplinary perspectives on emerging challenges, opportunities, and agenda for research, practice and policy. International Journal of Information Management, 57, p.101994.
Dwivedi, Y.K., Pandey, N., Currie, W. and Micu, A., (2024). Leveraging ChatGPT and other generative artificial intelligence (AI)-based applications in the hospitality and tourism industry: practices, challenges and research agenda. International Journal of Contemporary Hospitality Management, 36(1), pp.1-12.
Einola, K. and Khoreva, V., (2023). Best friend or broken tool? Exploring the co‐existence of humans and artificial intelligence in the workplace ecosystem. Human Resource Management, 62(1), pp.117-135.
Firican, G. (2022) The history of Big Data, LightsOnData. Available at: https://www.lightsondata.com/the-history-of-big-data/#:~:text=Some%20argue%20that%20it%20has,the%20O’Reilly%20Media%20group. (Accessed: 16 February 2024).
Frey, C.B. and Osborne, M., (2023). Generative AI and the Future of Work: A Reappraisal. Brown Journal of World Affairs, pp.1-12.
Fu, Q., Abdul Rahman, A.A., Jiang, H., Abbas, J. and Comite, U., (2022). Sustainable supply chain and business performance: The impact of strategy, network design, information systems, and organizational structure. Sustainability, 14(3), p.1080.
Grossman, M. and Gerrand, V., (2021). Terrorism confidential: Ethics, primary data, and the construction of “necessary fictions”. Terrorism and political violence, 33(2), pp.242-256.
Guo, J., Mallinson, D.J., Ortiz, S.E. and Iulo, L.D., (2024). Collaborative governance challenges in energy efficiency and conservation: The case of Pennsylvania. Utilities Policy, 87, p.101721.
Gupta, A. and Gupta, N., (2022). Research methodology. SBPD Publications.
Hangleiter, D. and Eisert, J., (2023). Computational advantage of quantum random sampling. Reviews of Modern Physics, 95(3), p.035001.
Hongal, P. and Kinange, U., (2020). A study on talent management and its impact on organization performance-an empirical review. International Journal of Engineering and Management Research, 10.
Howes, C.S. and Taylor, R.W., (2020), January. Building Technical, Commercial and Soft Skills in Evolving Organizations. In International Petroleum Technology Conference (p. D013S019R003). IPTC.
Imran, F., Shahzad, K., Butt, A. and Kantola, J., (2021). Digital transformation of industrial organizations: Toward an integrated framework. Journal of change management, 21(4), pp.451-479.
Jaiswal, A., Arun, C.J. and Varma, A., (2022). Rebooting employees: Upskilling for artificial intelligence in multinational corporations. The International Journal of Human Resource Management, 33(6), pp.1179-1208.
Johri, P., Singh, J.N., Sharma, A. and Rastogi, D., (2021), December. Sustainability of coexistence of humans and machines: an evolution of industry 5.0 from industry 4.0. In 2021 10th International Conference on System Modeling & Advancement in Research Trends (SMART) (pp. 410-414). IEEE.
Kamal, S.A., Shafiq, M. and Kakria, P., (2020). Investigating acceptance of telemedicine services through an extended technology acceptance model (TAM). Technology in Society, 60, p.101212.
Khan, O., Daddi, T. and Iraldo, F., (2020). Microfoundations of dynamic capabilities: Insights from circular economy business cases. Business Strategy and the Environment, 29(3), pp.1479-1493.
Kuknor, S.C. and Bhattacharya, S., (2022). Inclusive leadership: new age leadership to foster organizational inclusion. European Journal of Training and Development, 46(9), pp.771-797.
Kumatongo, B. and Muzata, K.K., (2021). Research paradigms and designs with their application in education. Journal of Lexicography and Terminology (Online ISSN 2664-0899. Print ISSN 2517-9306)., 5(1), pp.16-32.
Leavy, S., (2018), May. Gender bias in artificial intelligence: The need for diversity and gender theory in machine learning. In Proceedings of the 1st international workshop on gender equality in software engineering (pp. 14-16).
Liu, S. and Koslicki, D., (2023). Connecting Syncmers to FracMinHash: similarities and advantages. bioRxiv, pp.2023-11.
Lott, Y., (2020). Does flexibility help employees switch off from work? Flexible working-time arrangements and cognitive work-to-home spillover for women and men in Germany. Social Indicators Research, 151(2), pp.471-494.
Lyu, W. and Liu, J., (2021). Soft skills, hard skills: What matters most? Evidence from job postings. Applied Energy, 300, p.117307.
Maalouf, G.Y., (2023). The Role of Servant Leadership Style in Improving Innovation in Higher Education Institutions. International Journal of Professional Business Review, 8(9), pp.e01787-e01787.
Makala, B. and Bakovic, T., (2020). Artificial intelligence in the power sector.
Maksimovic, J. and Evtimov, J., (2023). Positivism and post-positivism as the basis of quantitative research in pedagogy. Research in Pedagogy, 13(1), pp.208-218.
Malazdrewicz, S., Ostrowski, K.A. and Sadowski, Ł., (2022). Large Panel System Technology in the Second Half of the Twentieth Century—Literature Review, Recycling Possibilities and Research Gaps. Buildings, 12(11), p.1822.
Mann, C., Garcia-Martin, M., Raymond, C.M., Shaw, B.J. and Plieninger, T., (2018). The potential for integrated landscape management to fulfil Europe’s commitments to the Sustainable Development Goals. Landscape and urban planning, 177, pp.75-82.
Mannuru, N.R., Shahriar, S., Teel, Z.A., Wang, T., Lund, B.D., Tijani, S., Pohboon, C.O., Agbaji, D., Alhassan, J., Galley, J. and Kousari, R., (2023). Artificial intelligence in developing countries: The impact of generative artificial intelligence (AI) technologies for development. Information Development, p.02666669231200628.
Mardiana, S., (2020). Modifying Research Onion for Information Systems Research. Solid State Technology, 63(4), pp.5304-5313.
Marion, T.J. and Fixson, S.K., (2021). The transformation of the innovation process: How digital tools are changing work, collaboration, and organizations in new product development. Journal of Product Innovation Management, 38(1), pp.192-215.
McDonald, R.I., Mansur, A.V., Ascensão, F., Colbert, M.L., Crossman, K., Elmqvist, T., Gonzalez, A., Güneralp, B., Haase, D., Hamann, M. and Hillel, O., (2020). Research gaps in knowledge of the impact of urban growth on biodiversity. Nature Sustainability, 3(1), pp.16-24.
Meng, B. and Choi, K., (2021). Employees’ sabotage formation in upscale hotels based on conservation of resources theory (COR): antecedents and strategies of attachment intervention. International Journal of Contemporary Hospitality Management, 33(3), pp.790-807.
Mishra, S.B. and Alok, S., (2022). Handbook of research methodology.
Mugira, A., (2022). Leadership Perspective Employee Satisfaction Analysis. AKADEMIK: Jurnal Mahasiswa Humanis, 2(3), pp.127-135.
Mukherjee, S.P., (2019). A guide to research methodology: An overview of research problems, tasks, and methods.
Mukumbang, F.C., (2023). Retroductive theorizing: a contribution of critical realism to mixed methods research. Journal of Mixed Methods Research, 17(1), pp.93-114.
Münch, C., Marx, E., Benz, L., Hartmann, E. and Matzner, M., (2022). Capabilities of digital servitization: Evidence from the socio-technical systems theory. Technological Forecasting and Social Change, 176, p.121361.
Musa, A., (2023). Revolutionizing Oil and Gas Industries with Artificial Intelligence Technology.
Nair, S.S. and Prem, S.S., (2020). A framework for mixed-method research. Shanlax International Journal of Management, 8(2), pp.45-53.
Ooi, K.B., Tan, G.W.H., Al-Emran, M., Al-Sharafi, M.A., Capatina, A., Chakraborty, A., Dwivedi, Y.K., Huang, T.L., Kar, A.K., Lee, V.H. and Loh, X.M., (2023). The potential of generative artificial intelligence across disciplines: Perspectives and future directions. Journal of Computer Information Systems, pp.1-32.
Park, D., Bahrudin, F. and Han, J., (2020). Circular reasoning for the evolution of research through a strategic construction of research methodologies. International Journal of Quantitative and Qualitative Research Methods.
Park, Y.S., Konge, L. and Artino, A.R., (2020). The positivism paradigm of research. Academic medicine, 95(5), pp.690-694.
Pillai, A.A. and Kaushal, U., (2020). Research Methodology-An Introduction To Literary Studies. Central Asian Journal of Literature, Philosophy and Culture, 1(1), pp.1-11.
Pradeep, R.M.M. and Wijesekera, N.T.S., (2022), September. Development of HydroGIS Model Development Framework: Research Methodological Perspectives. In Proceedings of the 15th International Research Conference, Ratmalana, Sri Lanka (pp. 29-30).
Rafique, H., Almagrabi, A.O., Shamim, A., Anwar, F., and Bashir, A.K., (2020). Investigating the acceptance of mobile library applications with an extended technology acceptance model (TAM). Computers & Education, 145, p.103732.
Rashid, A., Rasheed, R., Amirah, N.A., Yusof, Y., Khan, S., and Agha, A.A., (2021). A Quantitative Perspective of Systematic Research: Easy and Step-by-Step Initial Guidelines. Turkish Online Journal of Qualitative Inquiry, 12(9).
Singh, B., (2023). Federated learning for envision future trajectory smart transport system for climate preservation and smart green planet: Insights into global governance and SDG-9 (Industry, Innovation and Infrastructure). National Journal of Environmental Law, 6(2), pp.6-17.
Smith, J.G., Lindsay, J.B., Smith, J.G. and Lindsay, J.B., (2014). Mentoring and coaching. Beyond Inclusion: Worklife Interconnectedness, Energy, and Resilience in Organizations, pp.81-99.
Sony, M. and Naik, S., (2020). Industry 4.0 integration with socio-technical systems theory: A systematic review and proposed theoretical model. Technology in society, 61, p.101248.
Sowa, K., Przegalinska, A. and Ciechanowski, L., (2021). Cobots in knowledge work: Human–AI collaboration in managerial professions. Journal of Business Research, 125, pp.135-142.
Srivastava, M., (2020). Cooperative learning in design studios: a pedagogy for net-positive performance. Buildings and Cities, 1(1).
Stahl, G.K., Brewster, C.J., Collings, D.G. and Hajro, A., (2020). Enhancing the role of human resource management in corporate sustainability and social responsibility: A multi-stakeholder, multidimensional approach to HRM. Human Resource Management Review, 30(3), p.100708.
Stoeger, H., Balestrini, D.P. and Ziegler, A., (2021). Key issues in professionalizing mentoring practices. Annals of the New York Academy of Sciences, 1483(1), pp.5-18.
Sulich, A. and Rutkowska, M., (2020). Green jobs, definitional issues, and the employment of young people: An analysis of three European Union countries. Journal of environmental management, 262, p.110314.
Suri, H., (2020). Ethical considerations of conducting systematic reviews in educational research. Systematic reviews in educational research: Methodology, perspectives and application, pp.41-54.
Susanto, P.C. and Sawitri, N.N., (2022). Coaching, Mentoring, Leadership Transformation and Employee Engagement: A Review of the Literature. Dinasti International Journal Of Education Management And Social Science, 4(2), pp.297-308.
Teng, Z.L., Guo, C., Zhao, Q. and Mubarik, M.S., (2023). Antecedents of green process innovation adoption: An AHP analysis of China’s gas sector. Resources Policy, 85, p.103959.
Tschang, F.T. and Almirall, E., (2021). Artificial intelligence as augmenting automation: Implications for employment. Academy of Management Perspectives, 35(4), pp.642-659.
Urbano, D., Audretsch, D., Aparicio, S. and Noguera, M., (2020). Does entrepreneurial activity matter for economic growth in developing countries? The role of the institutional environment. International Entrepreneurship and Management Journal, 16, pp.1065-1099.
Vlačić, B., Corbo, L., e Silva, S.C. and Dabić, M., (2021). The evolving role of artificial intelligence in marketing: A review and research agenda. Journal of Business Research, 128, pp.187-203.
Vrontis, D., Christofi, M., Pereira, V., Tarba, S., Makrides, A. and Trichina, E., (2022). Artificial intelligence, robotics, advanced technologies, and human resource management: a systematic review. The International Journal of Human Resource Management, 33(6), pp.1237-1266.
Walkowiak, E. and MacDonald, T., (2023). Generative AI and the Workforce: What Are the Risks?.Available at SSRN.
Warsah, I., Morganna, R., Uyun, M., Afandi, M. and Hamengkubuwono, H., (2021). The impact of collaborative learning on learners’ critical thinking skills. International Journal of Instruction, 14(2), pp.443-460.
Zirar, A., Ali, S.I. and Islam, N., (2023). Worker and workplace Artificial Intelligence (AI) coexistence: Emerging themes and research agenda. Technovation, 124, p.102747.